Note
Click here to download the full example code
Spatial Transformer Networks Tutorial¶
Created On: Nov 08, 2017 | Last Updated: Jan 19, 2024 | Last Verified: Nov 05, 2024
Author: Ghassen HAMROUNI
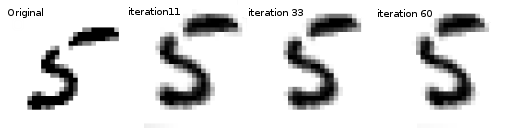
In this tutorial, you will learn how to augment your network using a visual attention mechanism called spatial transformer networks. You can read more about the spatial transformer networks in the DeepMind paper
Spatial transformer networks are a generalization of differentiable attention to any spatial transformation. Spatial transformer networks (STN for short) allow a neural network to learn how to perform spatial transformations on the input image in order to enhance the geometric invariance of the model. For example, it can crop a region of interest, scale and correct the orientation of an image. It can be a useful mechanism because CNNs are not invariant to rotation and scale and more general affine transformations.
One of the best things about STN is the ability to simply plug it into any existing CNN with very little modification.
# License: BSD
# Author: Ghassen Hamrouni
import torch
import torch.nn as nn
import torch.nn.functional as F
import torch.optim as optim
import torchvision
from torchvision import datasets, transforms
import matplotlib.pyplot as plt
import numpy as np
plt.ion() # interactive mode
<contextlib.ExitStack object at 0x7f9c7a6edde0>
Loading the data¶
In this post we experiment with the classic MNIST dataset. Using a standard convolutional network augmented with a spatial transformer network.
from six.moves import urllib
opener = urllib.request.build_opener()
opener.addheaders = [('User-agent', 'Mozilla/5.0')]
urllib.request.install_opener(opener)
device = torch.device("cuda" if torch.cuda.is_available() else "cpu")
# Training dataset
train_loader = torch.utils.data.DataLoader(
datasets.MNIST(root='.', train=True, download=True,
transform=transforms.Compose([
transforms.ToTensor(),
transforms.Normalize((0.1307,), (0.3081,))
])), batch_size=64, shuffle=True, num_workers=4)
# Test dataset
test_loader = torch.utils.data.DataLoader(
datasets.MNIST(root='.', train=False, transform=transforms.Compose([
transforms.ToTensor(),
transforms.Normalize((0.1307,), (0.3081,))
])), batch_size=64, shuffle=True, num_workers=4)
Downloading http://yann.lecun.com/exdb/mnist/train-images-idx3-ubyte.gz
Failed to download (trying next):
HTTP Error 403: Forbidden
Downloading https://ossci-datasets.s3.amazonaws.com/mnist/train-images-idx3-ubyte.gz
Downloading https://ossci-datasets.s3.amazonaws.com/mnist/train-images-idx3-ubyte.gz to ./MNIST/raw/train-images-idx3-ubyte.gz
0%| | 0.00/9.91M [00:00<?, ?B/s]
100%|##########| 9.91M/9.91M [00:00<00:00, 129MB/s]
Extracting ./MNIST/raw/train-images-idx3-ubyte.gz to ./MNIST/raw
Downloading http://yann.lecun.com/exdb/mnist/train-labels-idx1-ubyte.gz
Failed to download (trying next):
HTTP Error 403: Forbidden
Downloading https://ossci-datasets.s3.amazonaws.com/mnist/train-labels-idx1-ubyte.gz
Downloading https://ossci-datasets.s3.amazonaws.com/mnist/train-labels-idx1-ubyte.gz to ./MNIST/raw/train-labels-idx1-ubyte.gz
0%| | 0.00/28.9k [00:00<?, ?B/s]
100%|##########| 28.9k/28.9k [00:00<00:00, 37.4MB/s]
Extracting ./MNIST/raw/train-labels-idx1-ubyte.gz to ./MNIST/raw
Downloading http://yann.lecun.com/exdb/mnist/t10k-images-idx3-ubyte.gz
Failed to download (trying next):
HTTP Error 403: Forbidden
Downloading https://ossci-datasets.s3.amazonaws.com/mnist/t10k-images-idx3-ubyte.gz
Downloading https://ossci-datasets.s3.amazonaws.com/mnist/t10k-images-idx3-ubyte.gz to ./MNIST/raw/t10k-images-idx3-ubyte.gz
0%| | 0.00/1.65M [00:00<?, ?B/s]
100%|##########| 1.65M/1.65M [00:00<00:00, 139MB/s]
Extracting ./MNIST/raw/t10k-images-idx3-ubyte.gz to ./MNIST/raw
Downloading http://yann.lecun.com/exdb/mnist/t10k-labels-idx1-ubyte.gz
Failed to download (trying next):
HTTP Error 403: Forbidden
Downloading https://ossci-datasets.s3.amazonaws.com/mnist/t10k-labels-idx1-ubyte.gz
Downloading https://ossci-datasets.s3.amazonaws.com/mnist/t10k-labels-idx1-ubyte.gz to ./MNIST/raw/t10k-labels-idx1-ubyte.gz
0%| | 0.00/4.54k [00:00<?, ?B/s]
100%|##########| 4.54k/4.54k [00:00<00:00, 19.9MB/s]
Extracting ./MNIST/raw/t10k-labels-idx1-ubyte.gz to ./MNIST/raw
Depicting spatial transformer networks¶
Spatial transformer networks boils down to three main components :
The localization network is a regular CNN which regresses the transformation parameters. The transformation is never learned explicitly from this dataset, instead the network learns automatically the spatial transformations that enhances the global accuracy.
The grid generator generates a grid of coordinates in the input image corresponding to each pixel from the output image.
The sampler uses the parameters of the transformation and applies it to the input image.
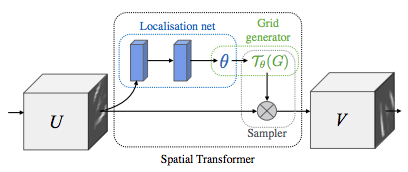
Note
We need the latest version of PyTorch that contains affine_grid and grid_sample modules.
class Net(nn.Module):
def __init__(self):
super(Net, self).__init__()
self.conv1 = nn.Conv2d(1, 10, kernel_size=5)
self.conv2 = nn.Conv2d(10, 20, kernel_size=5)
self.conv2_drop = nn.Dropout2d()
self.fc1 = nn.Linear(320, 50)
self.fc2 = nn.Linear(50, 10)
# Spatial transformer localization-network
self.localization = nn.Sequential(
nn.Conv2d(1, 8, kernel_size=7),
nn.MaxPool2d(2, stride=2),
nn.ReLU(True),
nn.Conv2d(8, 10, kernel_size=5),
nn.MaxPool2d(2, stride=2),
nn.ReLU(True)
)
# Regressor for the 3 * 2 affine matrix
self.fc_loc = nn.Sequential(
nn.Linear(10 * 3 * 3, 32),
nn.ReLU(True),
nn.Linear(32, 3 * 2)
)
# Initialize the weights/bias with identity transformation
self.fc_loc[2].weight.data.zero_()
self.fc_loc[2].bias.data.copy_(torch.tensor([1, 0, 0, 0, 1, 0], dtype=torch.float))
# Spatial transformer network forward function
def stn(self, x):
xs = self.localization(x)
xs = xs.view(-1, 10 * 3 * 3)
theta = self.fc_loc(xs)
theta = theta.view(-1, 2, 3)
grid = F.affine_grid(theta, x.size())
x = F.grid_sample(x, grid)
return x
def forward(self, x):
# transform the input
x = self.stn(x)
# Perform the usual forward pass
x = F.relu(F.max_pool2d(self.conv1(x), 2))
x = F.relu(F.max_pool2d(self.conv2_drop(self.conv2(x)), 2))
x = x.view(-1, 320)
x = F.relu(self.fc1(x))
x = F.dropout(x, training=self.training)
x = self.fc2(x)
return F.log_softmax(x, dim=1)
model = Net().to(device)
Training the model¶
Now, let’s use the SGD algorithm to train the model. The network is learning the classification task in a supervised way. In the same time the model is learning STN automatically in an end-to-end fashion.
optimizer = optim.SGD(model.parameters(), lr=0.01)
def train(epoch):
model.train()
for batch_idx, (data, target) in enumerate(train_loader):
data, target = data.to(device), target.to(device)
optimizer.zero_grad()
output = model(data)
loss = F.nll_loss(output, target)
loss.backward()
optimizer.step()
if batch_idx % 500 == 0:
print('Train Epoch: {} [{}/{} ({:.0f}%)]\tLoss: {:.6f}'.format(
epoch, batch_idx * len(data), len(train_loader.dataset),
100. * batch_idx / len(train_loader), loss.item()))
#
# A simple test procedure to measure the STN performances on MNIST.
#
def test():
with torch.no_grad():
model.eval()
test_loss = 0
correct = 0
for data, target in test_loader:
data, target = data.to(device), target.to(device)
output = model(data)
# sum up batch loss
test_loss += F.nll_loss(output, target, size_average=False).item()
# get the index of the max log-probability
pred = output.max(1, keepdim=True)[1]
correct += pred.eq(target.view_as(pred)).sum().item()
test_loss /= len(test_loader.dataset)
print('\nTest set: Average loss: {:.4f}, Accuracy: {}/{} ({:.0f}%)\n'
.format(test_loss, correct, len(test_loader.dataset),
100. * correct / len(test_loader.dataset)))
Visualizing the STN results¶
Now, we will inspect the results of our learned visual attention mechanism.
We define a small helper function in order to visualize the transformations while training.
def convert_image_np(inp):
"""Convert a Tensor to numpy image."""
inp = inp.numpy().transpose((1, 2, 0))
mean = np.array([0.485, 0.456, 0.406])
std = np.array([0.229, 0.224, 0.225])
inp = std * inp + mean
inp = np.clip(inp, 0, 1)
return inp
# We want to visualize the output of the spatial transformers layer
# after the training, we visualize a batch of input images and
# the corresponding transformed batch using STN.
def visualize_stn():
with torch.no_grad():
# Get a batch of training data
data = next(iter(test_loader))[0].to(device)
input_tensor = data.cpu()
transformed_input_tensor = model.stn(data).cpu()
in_grid = convert_image_np(
torchvision.utils.make_grid(input_tensor))
out_grid = convert_image_np(
torchvision.utils.make_grid(transformed_input_tensor))
# Plot the results side-by-side
f, axarr = plt.subplots(1, 2)
axarr[0].imshow(in_grid)
axarr[0].set_title('Dataset Images')
axarr[1].imshow(out_grid)
axarr[1].set_title('Transformed Images')
for epoch in range(1, 20 + 1):
train(epoch)
test()
# Visualize the STN transformation on some input batch
visualize_stn()
plt.ioff()
plt.show()
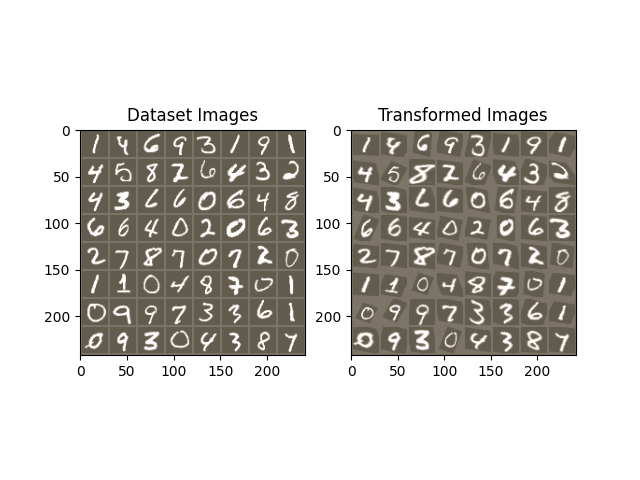
/usr/local/lib/python3.10/dist-packages/torch/nn/functional.py:4969: UserWarning:
Default grid_sample and affine_grid behavior has changed to align_corners=False since 1.3.0. Please specify align_corners=True if the old behavior is desired. See the documentation of grid_sample for details.
/usr/local/lib/python3.10/dist-packages/torch/nn/functional.py:4902: UserWarning:
Default grid_sample and affine_grid behavior has changed to align_corners=False since 1.3.0. Please specify align_corners=True if the old behavior is desired. See the documentation of grid_sample for details.
Train Epoch: 1 [0/60000 (0%)] Loss: 2.315648
Train Epoch: 1 [32000/60000 (53%)] Loss: 1.070642
/usr/local/lib/python3.10/dist-packages/torch/nn/_reduction.py:51: UserWarning:
size_average and reduce args will be deprecated, please use reduction='sum' instead.
Test set: Average loss: 0.2695, Accuracy: 9258/10000 (93%)
Train Epoch: 2 [0/60000 (0%)] Loss: 0.601887
Train Epoch: 2 [32000/60000 (53%)] Loss: 0.316032
Test set: Average loss: 0.2038, Accuracy: 9406/10000 (94%)
Train Epoch: 3 [0/60000 (0%)] Loss: 0.376618
Train Epoch: 3 [32000/60000 (53%)] Loss: 0.211189
Test set: Average loss: 0.1698, Accuracy: 9458/10000 (95%)
Train Epoch: 4 [0/60000 (0%)] Loss: 0.455998
Train Epoch: 4 [32000/60000 (53%)] Loss: 0.138938
Test set: Average loss: 0.1039, Accuracy: 9696/10000 (97%)
Train Epoch: 5 [0/60000 (0%)] Loss: 0.186056
Train Epoch: 5 [32000/60000 (53%)] Loss: 0.181154
Test set: Average loss: 0.1376, Accuracy: 9559/10000 (96%)
Train Epoch: 6 [0/60000 (0%)] Loss: 0.161735
Train Epoch: 6 [32000/60000 (53%)] Loss: 0.099665
Test set: Average loss: 0.0704, Accuracy: 9793/10000 (98%)
Train Epoch: 7 [0/60000 (0%)] Loss: 0.126997
Train Epoch: 7 [32000/60000 (53%)] Loss: 0.283283
Test set: Average loss: 0.1047, Accuracy: 9700/10000 (97%)
Train Epoch: 8 [0/60000 (0%)] Loss: 0.238581
Train Epoch: 8 [32000/60000 (53%)] Loss: 0.083868
Test set: Average loss: 0.0726, Accuracy: 9762/10000 (98%)
Train Epoch: 9 [0/60000 (0%)] Loss: 0.116012
Train Epoch: 9 [32000/60000 (53%)] Loss: 0.126550
Test set: Average loss: 0.0699, Accuracy: 9788/10000 (98%)
Train Epoch: 10 [0/60000 (0%)] Loss: 0.103091
Train Epoch: 10 [32000/60000 (53%)] Loss: 0.240384
Test set: Average loss: 0.0574, Accuracy: 9835/10000 (98%)
Train Epoch: 11 [0/60000 (0%)] Loss: 0.130547
Train Epoch: 11 [32000/60000 (53%)] Loss: 0.083143
Test set: Average loss: 0.0648, Accuracy: 9809/10000 (98%)
Train Epoch: 12 [0/60000 (0%)] Loss: 0.121329
Train Epoch: 12 [32000/60000 (53%)] Loss: 0.187620
Test set: Average loss: 0.0559, Accuracy: 9843/10000 (98%)
Train Epoch: 13 [0/60000 (0%)] Loss: 0.091729
Train Epoch: 13 [32000/60000 (53%)] Loss: 0.099912
Test set: Average loss: 0.0532, Accuracy: 9843/10000 (98%)
Train Epoch: 14 [0/60000 (0%)] Loss: 0.042855
Train Epoch: 14 [32000/60000 (53%)] Loss: 0.156960
Test set: Average loss: 0.0505, Accuracy: 9850/10000 (98%)
Train Epoch: 15 [0/60000 (0%)] Loss: 0.036390
Train Epoch: 15 [32000/60000 (53%)] Loss: 0.095055
Test set: Average loss: 0.0454, Accuracy: 9864/10000 (99%)
Train Epoch: 16 [0/60000 (0%)] Loss: 0.086283
Train Epoch: 16 [32000/60000 (53%)] Loss: 0.230836
Test set: Average loss: 0.0480, Accuracy: 9855/10000 (99%)
Train Epoch: 17 [0/60000 (0%)] Loss: 0.297770
Train Epoch: 17 [32000/60000 (53%)] Loss: 0.154699
Test set: Average loss: 0.0537, Accuracy: 9840/10000 (98%)
Train Epoch: 18 [0/60000 (0%)] Loss: 0.094822
Train Epoch: 18 [32000/60000 (53%)] Loss: 0.092634
Test set: Average loss: 0.0428, Accuracy: 9870/10000 (99%)
Train Epoch: 19 [0/60000 (0%)] Loss: 0.050314
Train Epoch: 19 [32000/60000 (53%)] Loss: 0.094214
Test set: Average loss: 0.0431, Accuracy: 9861/10000 (99%)
Train Epoch: 20 [0/60000 (0%)] Loss: 0.039528
Train Epoch: 20 [32000/60000 (53%)] Loss: 0.225297
Test set: Average loss: 0.0435, Accuracy: 9875/10000 (99%)
Total running time of the script: ( 2 minutes 27.177 seconds)