torchx.schedulers¶
TorchX Schedulers define plugins to existing schedulers. Used with the runner, they submit components as jobs onto the respective scheduler backends. TorchX supports a few schedulers out-of-the-box. You can add your own by implementing .. py:class::torchx.schedulers and registering it in the entrypoint.
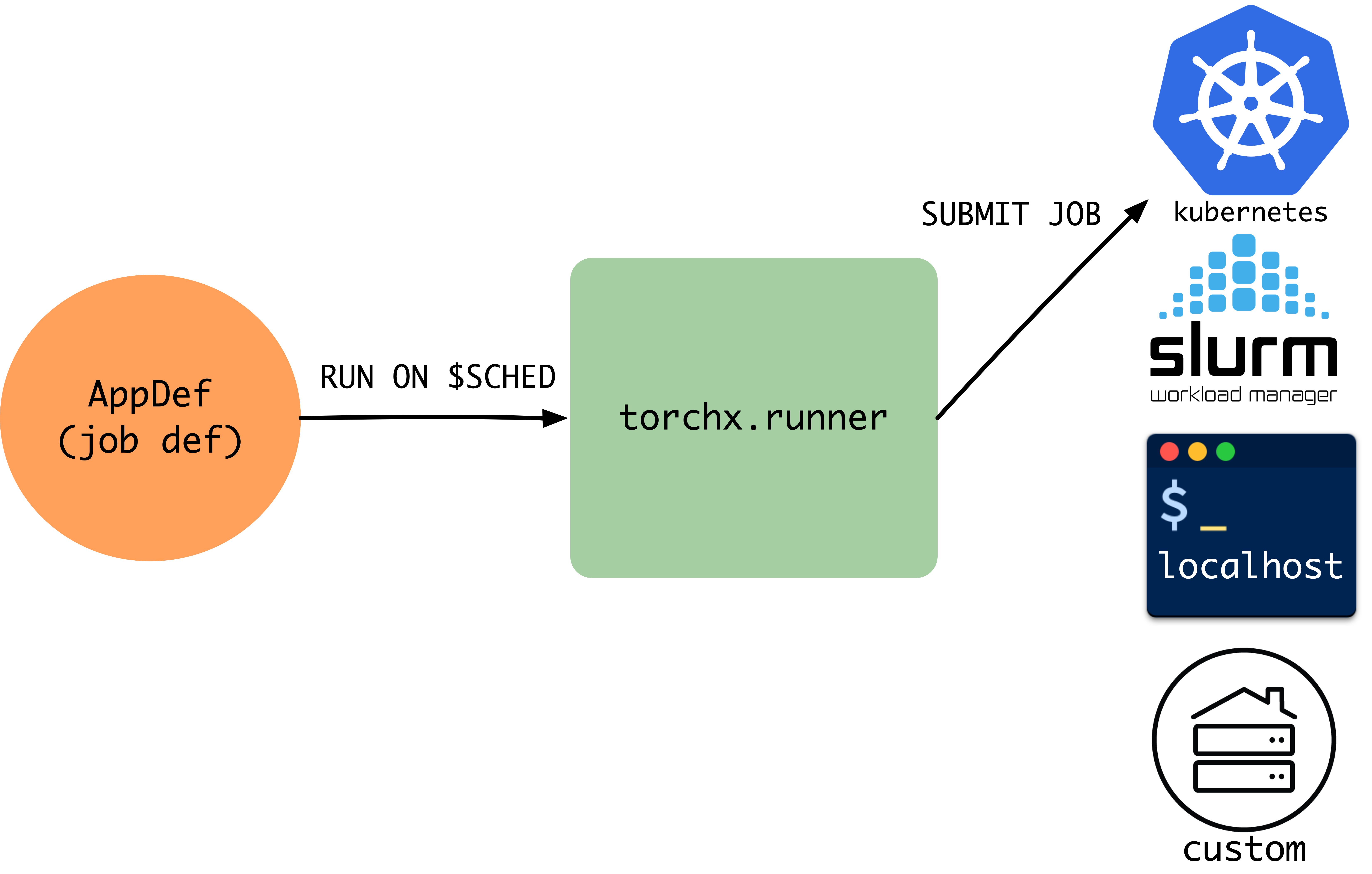
All Schedulers¶
Scheduler Functions¶
- torchx.schedulers.get_scheduler_factories() Dict[str, SchedulerFactory] [source]¶
get_scheduler_factories returns all the available schedulers names and the method to instantiate them.
The first scheduler in the dictionary is used as the default scheduler.
Scheduler Classes¶
- class torchx.schedulers.Scheduler(backend: str, session_name: str)[source]¶
An interface abstracting functionalities of a scheduler. Implementers need only implement those methods annotated with
@abc.abstractmethod
.- cancel(app_id: str) None [source]¶
Cancels/kills the application. This method is idempotent within the same thread and is safe to call on the same application multiple times. However when called from multiple threads/processes on the same app the exact semantics of this method depends on the idempotency guarantees of the underlying scheduler API.
Note
This method does not block for the application to reach a cancelled state. To ensure that the application reaches a terminal state use the
wait
API.
- close() None [source]¶
Only for schedulers that have local state! Closes the scheduler freeing any allocated resources. Once closed, the scheduler object is deemed to no longer be valid and any method called on the object results in undefined behavior.
This method should not raise exceptions and is allowed to be called multiple times on the same object.
Note
Override only for scheduler implementations that have local state (
torchx/schedulers/local_scheduler.py
). Schedulers simply wrapping a remote scheduler’s client need not implement this method.
- abstract describe(app_id: str) Optional[DescribeAppResponse] [source]¶
Describes the specified application.
- Returns:
AppDef description or
None
if the app does not exist.
- abstract list() List[ListAppResponse] [source]¶
For apps launched on the scheduler, this API returns a list of ListAppResponse objects each of which have app id and its status. Note: This API is in prototype phase and is subject to change.
- log_iter(app_id: str, role_name: str, k: int = 0, regex: Optional[str] = None, since: Optional[datetime] = None, until: Optional[datetime] = None, should_tail: bool = False, streams: Optional[Stream] = None) Iterable[str] [source]¶
Returns an iterator to the log lines of the
k``th replica of the ``role
. The iterator ends when all qualifying log lines have been read.If the scheduler supports time-based cursors fetching log lines for custom time ranges, then the
since
,until
fields are honored, otherwise they are ignored. Not specifyingsince
anduntil
is equivalent to getting all available log lines. If theuntil
is empty, then the iterator behaves liketail -f
, following the log output until the job reaches a terminal state.The exact definition of what constitutes a log is scheduler specific. Some schedulers may consider stderr or stdout as the log, others may read the logs from a log file.
Behaviors and assumptions:
Produces an undefined-behavior if called on an app that does not exist The caller should check that the app exists using
exists(app_id)
prior to calling this method.Is not stateful, calling this method twice with same parameters returns a new iterator. Prior iteration progress is lost.
Does not always support log-tailing. Not all schedulers support live log iteration (e.g. tailing logs while the app is running). Refer to the specific scheduler’s documentation for the iterator’s behavior.
- 3.1 If the scheduler supports log-tailing, it should be controlled
by
should_tail
parameter.
Does not guarantee log retention. It is possible that by the time this method is called, the underlying scheduler may have purged the log records for this application. If so this method raises an arbitrary exception.
If
should_tail
is True, the method only raises aStopIteration
exception when the accessible log lines have been fully exhausted and the app has reached a final state. For instance, if the app gets stuck and does not produce any log lines, then the iterator blocks until the app eventually gets killed (either via timeout or manually) at which point it raises aStopIteration
.If
should_tail
is False, the method raisesStopIteration
when there are no more logs.Need not be supported by all schedulers.
Some schedulers may support line cursors by supporting
__getitem__
(e.g.iter[50]
seeks to the 50th log line).- Whitespace is preserved, each new line should include
\n
. To support interactive progress bars the returned lines don’t need to include
\n
but should then be printed without a newline to correctly handle\r
carriage returns.
- Whitespace is preserved, each new line should include
- Parameters:
streams – The IO output streams to select. One of: combined, stdout, stderr. If the selected stream isn’t supported by the scheduler it will throw an ValueError.
- Returns:
An
Iterator
over log lines of the specified role replica- Raises:
NotImplementedError – if the scheduler does not support log iteration
- run_opts() runopts [source]¶
Returns the run configuration options expected by the scheduler. Basically a
--help
for therun
API.
- abstract schedule(dryrun_info: AppDryRunInfo) str [source]¶
Same as
submit
except that it takes anAppDryRunInfo
. Implementers are encouraged to implement this method rather than directly implementingsubmit
sincesubmit
can be trivially implemented by:dryrun_info = self.submit_dryrun(app, cfg) return schedule(dryrun_info)
- submit(app: AppDef, cfg: T, workspace: Optional[str] = None) str [source]¶
Submits the application to be run by the scheduler.
WARNING: Mostly used for tests. Users should prefer to use the TorchX runner instead.
- Returns:
The application id that uniquely identifies the submitted app.
- submit_dryrun(app: AppDef, cfg: T) AppDryRunInfo [source]¶
Rather than submitting the request to run the app, returns the request object that would have been submitted to the underlying service. The type of the request object is scheduler dependent. This method can be used to dry-run an application. Please refer to the scheduler implementation’s documentation regarding the actual return type.
- class torchx.schedulers.api.DescribeAppResponse(app_id: str = '<NOT_SET>', state: ~torchx.specs.api.AppState = AppState.UNSUBMITTED, num_restarts: int = -1, msg: str = '<NONE>', structured_error_msg: str = '<NONE>', ui_url: ~typing.Optional[str] = None, roles_statuses: ~typing.List[~torchx.specs.api.RoleStatus] = <factory>, roles: ~typing.List[~torchx.specs.api.Role] = <factory>)[source]¶
Response object returned by
Scheduler.describe(app)
API. Contains the status and description of the application as known by the scheduler. For some schedulers implementations this response object has necessary and sufficient information to recreate anAppDef
object. For these types of schedulers, the user can re-run()
the recreted application. Otherwise the user can only call non-creating methods (e.g.wait()
,status()
, etc).Since this class is a data class and contains many member variables we keep the usage simple and provide a no-args constructor and chose to access the member vars directly rather than provide accessors.
If scheduler returns arbitrary message, the
msg
field should be populated. If scheduler returns a structured json, thestructured_error_msg
field should be populated.
- class torchx.schedulers.api.ListAppResponse(app_id: str, state: AppState, app_handle: str = '<NOT_SET>')[source]¶
Response object returned by
scheduler.list()
andrunner.list()
APIs. Contains the app_id, app_handle and status of the application. App ID : The unique identifier that identifies apps submitted on the scheduler App handle: Identifier for apps run with torchx in a url format like {scheduler_backend}://{session_name}/{app_id}, which is created by the runner when it submits a job on a scheduler. Handle info in ListAppResponse is filled in byrunner.list()
. This handle can be used to further describe the app with torchx CLI or a torchx runner instance.Since this class is a data class with some member variables we keep the usage simple and chose to access the member vars directly rather than provide accessors.