Kubeflow Pipelines
TorchX provides an adapter to run TorchX components as part of Kubeflow Pipelines. See KubeFlow Pipelines Examples.
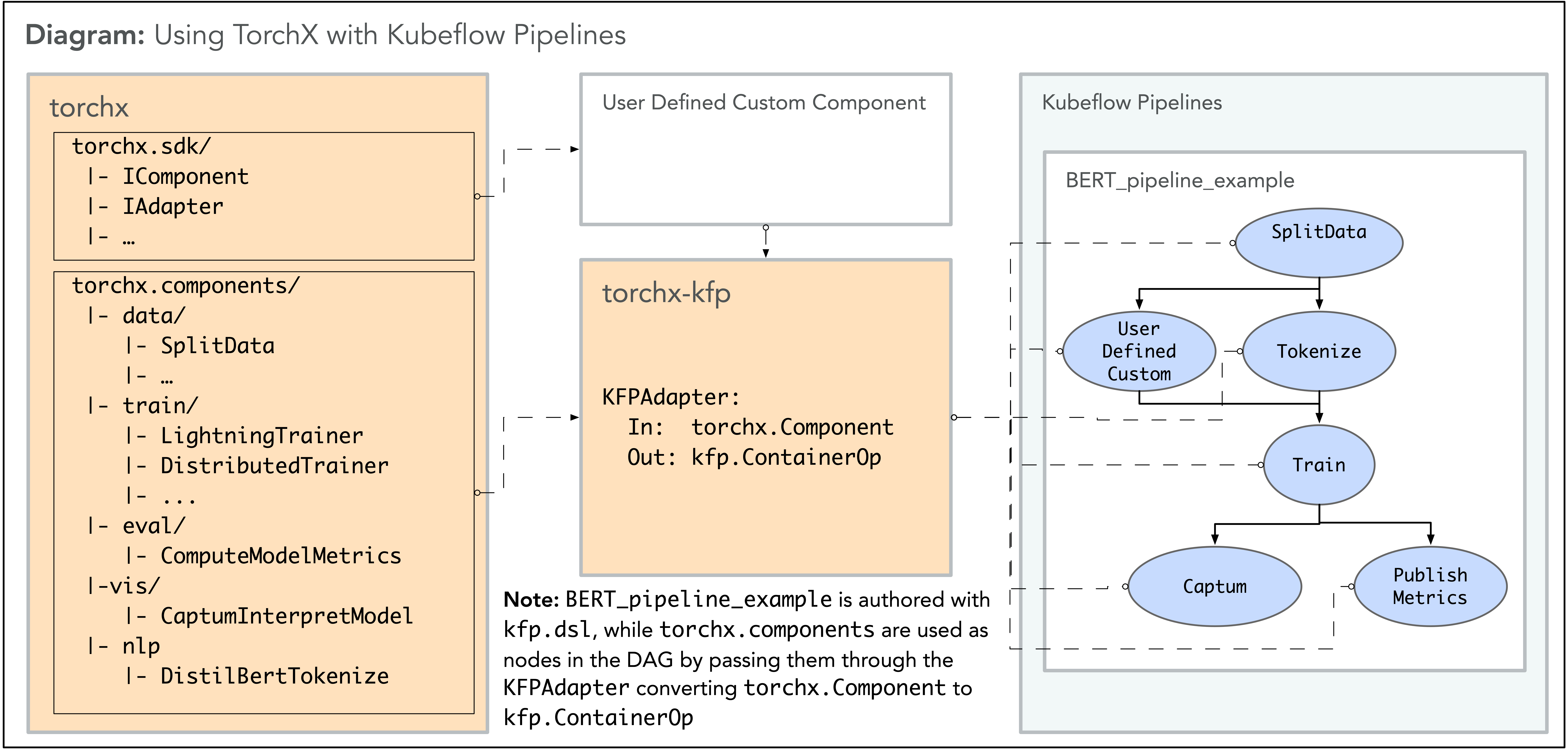
torchx.pipelines.kfp

This module contains adapters for converting TorchX components into KubeFlow Pipeline components.
The current KFP adapters only support single node (1 role and 1 replica) components.
- torchx.pipelines.kfp.adapter.container_from_app(app: AppDef, *args: object, ui_metadata: Optional[Mapping[str, object]] = None, **kwargs: object) ContainerOp [source]
container_from_app transforms the app into a KFP component and returns a corresponding ContainerOp instance.
See component_from_app for description on the arguments. Any unspecified arguments are passed through to the KFP container factory method.
>>> import kfp >>> from torchx import specs >>> from torchx.pipelines.kfp.adapter import container_from_app >>> app_def = specs.AppDef( ... name="trainer", ... roles=[specs.Role("trainer", image="foo:latest")], ... ) >>> def pipeline(): ... trainer = container_from_app(app_def) ... print(trainer) >>> kfp.compiler.Compiler().compile( ... pipeline_func=pipeline, ... package_path="/tmp/pipeline.yaml", ... ) {'ContainerOp': {... 'name': 'trainer-trainer', ...}}
- torchx.pipelines.kfp.adapter.resource_from_app(app: AppDef, queue: str, service_account: Optional[str] = None) ResourceOp [source]
resource_from_app generates a KFP ResourceOp from the provided app that uses the Volcano job scheduler on Kubernetes to run distributed apps. See https://volcano.sh/en/docs/ for more info on Volcano and how to install.
- Parameters:
app – The torchx AppDef to adapt.
queue – the Volcano queue to schedule the operator in.
>>> import kfp >>> from torchx import specs >>> from torchx.pipelines.kfp.adapter import resource_from_app >>> app_def = specs.AppDef( ... name="trainer", ... roles=[specs.Role("trainer", image="foo:latest", num_replicas=3)], ... ) >>> def pipeline(): ... trainer = resource_from_app(app_def, queue="test") ... print(trainer) >>> kfp.compiler.Compiler().compile( ... pipeline_func=pipeline, ... package_path="/tmp/pipeline.yaml", ... ) {'ResourceOp': {... 'name': 'trainer-0', ... 'name': 'trainer-1', ... 'name': 'trainer-2', ...}}
- torchx.pipelines.kfp.adapter.component_from_app(app: AppDef, ui_metadata: Optional[Mapping[str, object]] = None) ContainerFactory [source]
component_from_app takes in a TorchX component/AppDef and returns a KFP ContainerOp factory. This is equivalent to the kfp.components.load_component_from_* methods.
- Parameters:
app – The AppDef to generate a KFP container factory for.
ui_metadata – KFP UI Metadata to output so you can have model results show up in the UI. See https://www.kubeflow.org/docs/components/pipelines/legacy-v1/sdk/output-viewer/ for more info on the format.
>>> from torchx import specs >>> from torchx.pipelines.kfp.adapter import component_from_app >>> app_def = specs.AppDef( ... name="trainer", ... roles=[specs.Role("trainer", image="foo:latest")], ... ) >>> component_from_app(app_def) <function component_from_app...>
- torchx.pipelines.kfp.adapter.component_spec_from_app(app: AppDef) Tuple[str, Role] [source]
component_spec_from_app takes in a TorchX component and generates the yaml spec for it. Notably this doesn’t apply resources or port_maps since those must be applied at runtime which is why it returns the role spec as well.
>>> from torchx import specs >>> from torchx.pipelines.kfp.adapter import component_spec_from_app >>> app_def = specs.AppDef( ... name="trainer", ... roles=[specs.Role("trainer", image="foo:latest")], ... ) >>> component_spec_from_app(app_def) ('description: ...', Role(...))
- class torchx.pipelines.kfp.adapter.ContainerFactory(*args, **kwargs)[source]
ContainerFactory is a protocol that represents a function that when called produces a kfp.dsl.ContainerOp.