GPU video decoder/encoder¶
Author: Moto Hira
This tutorial shows how to use NVIDIA’s hardware video decoder (NVDEC) and encoder (NVENC) with TorchAudio.
Using hardware encoder/decoder improves the speed of loading and saving certain types of videos.
Using them in TorchAduio requires FFmpeg built with NVENC/NVDEC support. Google Colab has such FFmpeg pre-installed, so you can run this tutorial on Google Colab.
If you want to enable GPU decoding/encoding, please refer to Enabling GPU video decoder/encoder.
Update PyTorch and TorchAudio with nightly builds¶
Until PyTorch 2.0 is released, we need to use the nightly builds of PyTorch and TorchAudio.
[2]:
%%bash
pip uninstall -y -q torch torchaudio torchvision torchtext
pip install --progress-bar off --pre torch torchaudio --extra-index-url https://download.pytorch.org/whl/nightly/cu117 2> /dev/null
Looking in indexes: https://pypi.org/simple, https://us-python.pkg.dev/colab-wheels/public/simple/, https://download.pytorch.org/whl/nightly/cu117
Collecting torch
Downloading https://download.pytorch.org/whl/nightly/cu117/torch-2.0.0.dev20230209%2Bcu117-cp38-cp38-linux_x86_64.whl (1837.7 MB)
Collecting torchaudio
Downloading https://download.pytorch.org/whl/nightly/cu117/torchaudio-2.0.0.dev20230208%2Bcu117-cp38-cp38-linux_x86_64.whl (4.4 MB)
Requirement already satisfied: filelock in /usr/local/lib/python3.8/dist-packages (from torch) (3.9.0)
Collecting pytorch-triton==2.0.0+0d7e753227
Downloading https://download.pytorch.org/whl/nightly/pytorch_triton-2.0.0%2B0d7e753227-cp38-cp38-linux_x86_64.whl (18.7 MB)
Requirement already satisfied: typing-extensions in /usr/local/lib/python3.8/dist-packages (from torch) (4.4.0)
Requirement already satisfied: networkx in /usr/local/lib/python3.8/dist-packages (from torch) (3.0)
Requirement already satisfied: sympy in /usr/local/lib/python3.8/dist-packages (from torch) (1.7.1)
Requirement already satisfied: cmake in /usr/local/lib/python3.8/dist-packages (from pytorch-triton==2.0.0+0d7e753227->torch) (3.22.6)
Requirement already satisfied: mpmath>=0.19 in /usr/local/lib/python3.8/dist-packages (from sympy->torch) (1.2.1)
Installing collected packages: pytorch-triton, torch, torchaudio
Successfully installed pytorch-triton-2.0.0+0d7e753227 torch-2.0.0.dev20230209+cu117 torchaudio-2.0.0.dev20230208+cu117
Install third party libraries and download assets¶
[8]:
%%bash
pip3 install --progress-bar off boto3 2> /dev/null
Looking in indexes: https://pypi.org/simple, https://us-python.pkg.dev/colab-wheels/public/simple/
Collecting boto3
Downloading boto3-1.26.67-py3-none-any.whl (132 kB)
Collecting botocore<1.30.0,>=1.29.67
Downloading botocore-1.29.67-py3-none-any.whl (10.4 MB)
Collecting jmespath<2.0.0,>=0.7.1
Downloading jmespath-1.0.1-py3-none-any.whl (20 kB)
Collecting s3transfer<0.7.0,>=0.6.0
Downloading s3transfer-0.6.0-py3-none-any.whl (79 kB)
Requirement already satisfied: python-dateutil<3.0.0,>=2.1 in /usr/local/lib/python3.8/dist-packages (from botocore<1.30.0,>=1.29.67->boto3) (2.8.2)
Collecting urllib3<1.27,>=1.25.4
Downloading urllib3-1.26.14-py2.py3-none-any.whl (140 kB)
Requirement already satisfied: six>=1.5 in /usr/local/lib/python3.8/dist-packages (from python-dateutil<3.0.0,>=2.1->botocore<1.30.0,>=1.29.67->boto3) (1.15.0)
Installing collected packages: urllib3, jmespath, botocore, s3transfer, boto3
Attempting uninstall: urllib3
Found existing installation: urllib3 1.24.3
Uninstalling urllib3-1.24.3:
Successfully uninstalled urllib3-1.24.3
Successfully installed boto3-1.26.67 botocore-1.29.67 jmespath-1.0.1 s3transfer-0.6.0 urllib3-1.26.14
[10]:
%%bash
wget -q -O input.mp4 "https://download.pytorch.org/torchaudio/tutorial-assets/stream-api/NASAs_Most_Scientifically_Complex_Space_Observatory_Requires_Precision-MP4_small.mp4"
Check the available GPU¶
[1]:
%%bash
nvidia-smi
Thu Feb 9 15:54:05 2023
+-----------------------------------------------------------------------------+
| NVIDIA-SMI 510.47.03 Driver Version: 510.47.03 CUDA Version: 11.6 |
|-------------------------------+----------------------+----------------------+
| GPU Name Persistence-M| Bus-Id Disp.A | Volatile Uncorr. ECC |
| Fan Temp Perf Pwr:Usage/Cap| Memory-Usage | GPU-Util Compute M. |
| | | MIG M. |
|===============================+======================+======================|
| 0 Tesla T4 Off | 00000000:00:04.0 Off | 0 |
| N/A 58C P0 28W / 70W | 0MiB / 15360MiB | 0% Default |
| | | N/A |
+-------------------------------+----------------------+----------------------+
+-----------------------------------------------------------------------------+
| Processes: |
| GPU GI CI PID Type Process name GPU Memory |
| ID ID Usage |
|=============================================================================|
| No running processes found |
+-----------------------------------------------------------------------------+
Check FFmpeg installation¶
Let’s do a quick sanity check to confirm that the FFmpeg we built works.
[55]:
%%bash
ffmpeg -hide_banner -decoders | grep -i cuvid
V..... h264_cuvid Nvidia CUVID H264 decoder (codec h264)
V..... hevc_cuvid Nvidia CUVID HEVC decoder (codec hevc)
V..... mjpeg_cuvid Nvidia CUVID MJPEG decoder (codec mjpeg)
V..... mpeg1_cuvid Nvidia CUVID MPEG1VIDEO decoder (codec mpeg1video)
V..... mpeg2_cuvid Nvidia CUVID MPEG2VIDEO decoder (codec mpeg2video)
V..... mpeg4_cuvid Nvidia CUVID MPEG4 decoder (codec mpeg4)
V..... vc1_cuvid Nvidia CUVID VC1 decoder (codec vc1)
V..... vp8_cuvid Nvidia CUVID VP8 decoder (codec vp8)
V..... vp9_cuvid Nvidia CUVID VP9 decoder (codec vp9)
[56]:
%%bash
ffmpeg -hide_banner -encoders | grep -i nvenc
V..... h264_nvenc NVIDIA NVENC H.264 encoder (codec h264)
V..... nvenc NVIDIA NVENC H.264 encoder (codec h264)
V..... nvenc_h264 NVIDIA NVENC H.264 encoder (codec h264)
V..... nvenc_hevc NVIDIA NVENC hevc encoder (codec hevc)
V..... hevc_nvenc NVIDIA NVENC hevc encoder (codec hevc)
The following command fetches video from remote server, decode with NVDEC (cuvid) and re-encode with NVENC. If this command does not work, then there is an issue with FFmpeg installation, and TorchAudio would not be able to use them either.
[5]:
%%bash
ffmpeg -hide_banner -y -vsync 0 -hwaccel cuvid -hwaccel_output_format cuda -c:v h264_cuvid -resize 360x240 -i "https://download.pytorch.org/torchaudio/tutorial-assets/stream-api/NASAs_Most_Scientifically_Complex_Space_Observatory_Requires_Precision-MP4_small.mp4" -c:a copy -c:v h264_nvenc -b:v 5M test.mp4
Input #0, mov,mp4,m4a,3gp,3g2,mj2, from 'https://download.pytorch.org/torchaudio/tutorial-assets/stream-api/NASAs_Most_Scientifically_Complex_Space_Observatory_Requires_Precision-MP4_small.mp4':
Metadata:
major_brand : mp42
minor_version : 512
compatible_brands: mp42iso2avc1mp41
encoder : Lavf58.76.100
Duration: 00:03:26.04, start: 0.000000, bitrate: 1294 kb/s
Stream #0:0(eng): Video: h264 (High) (avc1 / 0x31637661), yuv420p(tv, bt709), 960x540 [SAR 1:1 DAR 16:9], 1156 kb/s, 29.97 fps, 29.97 tbr, 30k tbn, 59.94 tbc (default)
Metadata:
handler_name : ?Mainconcept Video Media Handler
Stream #0:1(eng): Audio: aac (LC) (mp4a / 0x6134706D), 48000 Hz, stereo, fltp, 128 kb/s (default)
Metadata:
handler_name : #Mainconcept MP4 Sound Media Handler
Stream mapping:
Stream #0:0 -> #0:0 (h264 (h264_cuvid) -> h264 (h264_nvenc))
Stream #0:1 -> #0:1 (copy)
Press [q] to stop, [?] for help
Output #0, mp4, to 'test.mp4':
Metadata:
major_brand : mp42
minor_version : 512
compatible_brands: mp42iso2avc1mp41
encoder : Lavf58.29.100
Stream #0:0(eng): Video: h264 (h264_nvenc) (Main) (avc1 / 0x31637661), cuda, 360x240 [SAR 1:1 DAR 3:2], q=-1--1, 5000 kb/s, 29.97 fps, 30k tbn, 29.97 tbc (default)
Metadata:
handler_name : ?Mainconcept Video Media Handler
encoder : Lavc58.54.100 h264_nvenc
Side data:
cpb: bitrate max/min/avg: 0/0/5000000 buffer size: 10000000 vbv_delay: -1
Stream #0:1(eng): Audio: aac (LC) (mp4a / 0x6134706D), 48000 Hz, stereo, fltp, 128 kb/s (default)
Metadata:
handler_name : #Mainconcept MP4 Sound Media Handler
frame= 6175 fps=1673 q=8.0 Lsize= 41278kB time=00:03:26.17 bitrate=1640.1kbits/s speed=55.9x
video:37869kB audio:3234kB subtitle:0kB other streams:0kB global headers:0kB muxing overhead: 0.424815%
Check the TorchAudio / FFmpeg integration¶
[6]:
import torch
import torchaudio
print(torch.__version__)
print(torchaudio.__version__)
from torchaudio.io import StreamReader, StreamWriter
2.0.0.dev20230209+cu117
2.0.0.dev20230208+cu117
[61]:
from torchaudio.utils import ffmpeg_utils
print("Library versions:")
print(ffmpeg_utils.get_versions())
print("\nBuild config:")
print(ffmpeg_utils.get_build_config())
print("\nDecoders:")
print([k for k in ffmpeg_utils.get_video_decoders().keys() if "cuvid" in k])
print("\nEncoders:")
print([k for k in ffmpeg_utils.get_video_encoders().keys() if "nvenc" in k])
Library versions:
{'libavutil': (56, 31, 100), 'libavcodec': (58, 54, 100), 'libavformat': (58, 29, 100), 'libavfilter': (7, 57, 100), 'libavdevice': (58, 8, 100)}
Build config:
--prefix=/usr --extra-version=0ubuntu0.1 --toolchain=hardened --libdir=/usr/lib/x86_64-linux-gnu --incdir=/usr/include/x86_64-linux-gnu --arch=amd64 --enable-gpl --disable-stripping --enable-avresample --disable-filter=resample --enable-avisynth --enable-gnutls --enable-ladspa --enable-libaom --enable-libass --enable-libbluray --enable-libbs2b --enable-libcaca --enable-libcdio --enable-libcodec2 --enable-libflite --enable-libfontconfig --enable-libfreetype --enable-libfribidi --enable-libgme --enable-libgsm --enable-libjack --enable-libmp3lame --enable-libmysofa --enable-libopenjpeg --enable-libopenmpt --enable-libopus --enable-libpulse --enable-librsvg --enable-librubberband --enable-libshine --enable-libsnappy --enable-libsoxr --enable-libspeex --enable-libssh --enable-libtheora --enable-libtwolame --enable-libvidstab --enable-libvorbis --enable-libvpx --enable-libwavpack --enable-libwebp --enable-libx265 --enable-libxml2 --enable-libxvid --enable-libzmq --enable-libzvbi --enable-lv2 --enable-omx --enable-openal --enable-opencl --enable-opengl --enable-sdl2 --enable-libdc1394 --enable-libdrm --enable-libiec61883 --enable-nvenc --enable-chromaprint --enable-frei0r --enable-libx264 --enable-shared
Decoders:
['h264_cuvid', 'hevc_cuvid', 'mjpeg_cuvid', 'mpeg1_cuvid', 'mpeg2_cuvid', 'mpeg4_cuvid', 'vc1_cuvid', 'vp8_cuvid', 'vp9_cuvid']
Encoders:
['h264_nvenc', 'nvenc', 'nvenc_h264', 'nvenc_hevc', 'hevc_nvenc']
Benchmarking GPU Encoding and Decoding¶
Now that FFmpeg and the resulting libraries are ready to use, we test NVDEC/NVENC with TorchAudio. For the basics of TorchAudio’s streaming APIs, please refer to Media I/O tutorials.
[7]:
import time
import matplotlib.pyplot as plt
import pandas as pd
pd.set_option('display.max_rows', None)
pd.set_option('display.max_columns', None)
Benchmark NVDEC with StreamReader
¶
First we test hardware decoding, and we fetch video from multiple locations (local file, network file, AWS S3) and use NVDEC to decod them.
[9]:
import boto3
from botocore import UNSIGNED
from botocore.config import Config
print(boto3.__version__)
1.26.67
First, we define the functions we’ll use for testing.
Funcion test_decode
decodes the given source from start to end, and it reports the elapsed time, and returns one image frmae as a sample.
[11]:
result = torch.zeros((4, 2))
samples = [[None, None] for _ in range(4)]
def test_decode(src, config, i_sample):
print("=" * 40)
print("* Configuration:", config)
print("* Source:", src)
print("=" * 40)
t0 = time.monotonic()
s = StreamReader(src)
s.add_video_stream(5, **config)
num_frames = 0
for i, (chunk, ) in enumerate(s.stream()):
if i == 0:
print(' - Chunk:', chunk.shape, chunk.device, chunk.dtype)
if i == i_sample:
sample = chunk[0]
num_frames += chunk.shape[0]
elapsed = time.monotonic() - t0
print()
print(f" - Processed {num_frames} frames.")
print(f" - Elapsed: {elapsed} seconds.")
print()
return elapsed, sample
Decode MP4 from local file¶
For the first test, we compare the time it takes for CPU and NVDEC to decode 250MB of MP4 video.
[12]:
local_src = "input.mp4"
i_sample = 520
CPU¶
[13]:
cpu_conf = {
"decoder": "h264", # CPU decoding
}
elapsed, sample = test_decode(local_src, cpu_conf, i_sample)
========================================
* Configuration: {'decoder': 'h264'}
* Source: input.mp4
========================================
- Chunk: torch.Size([5, 3, 540, 960]) cpu torch.uint8
- Processed 6175 frames.
- Elapsed: 43.65067997 seconds.
[14]:
result[0, 0] = elapsed
samples[0][0] = sample
CUDA¶
[15]:
cuda_conf = {
"decoder": "h264_cuvid", # Use CUDA HW decoder
"hw_accel": "cuda:0", # Then keep the memory on CUDA:0
}
elapsed, sample = test_decode(local_src, cuda_conf, i_sample)
========================================
* Configuration: {'decoder': 'h264_cuvid', 'hw_accel': 'cuda:0'}
* Source: input.mp4
========================================
- Chunk: torch.Size([5, 3, 540, 960]) cuda:0 torch.uint8
- Processed 6175 frames.
- Elapsed: 5.754925530000008 seconds.
[16]:
result[0, 1] = elapsed
samples[0][1] = sample
Decode MP4 from network¶
Let’s run the same test on the source retrieved via network on-the-fly.
[17]:
network_src = "https://download.pytorch.org/torchaudio/tutorial-assets/stream-api/NASAs_Most_Scientifically_Complex_Space_Observatory_Requires_Precision-MP4_small.mp4"
i_sample = 750
CPU¶
[18]:
elapsed, sample = test_decode(network_src, cpu_conf, i_sample)
========================================
* Configuration: {'decoder': 'h264'}
* Source: https://download.pytorch.org/torchaudio/tutorial-assets/stream-api/NASAs_Most_Scientifically_Complex_Space_Observatory_Requires_Precision-MP4_small.mp4
========================================
- Chunk: torch.Size([5, 3, 540, 960]) cpu torch.uint8
- Processed 6175 frames.
- Elapsed: 33.74701378400002 seconds.
[19]:
result[1, 0] = elapsed
samples[1][0] = sample
CUDA¶
[20]:
elapsed, sample = test_decode(network_src, cuda_conf, i_sample)
========================================
* Configuration: {'decoder': 'h264_cuvid', 'hw_accel': 'cuda:0'}
* Source: https://download.pytorch.org/torchaudio/tutorial-assets/stream-api/NASAs_Most_Scientifically_Complex_Space_Observatory_Requires_Precision-MP4_small.mp4
========================================
- Chunk: torch.Size([5, 3, 540, 960]) cuda:0 torch.uint8
- Processed 6175 frames.
- Elapsed: 15.769149663000007 seconds.
[21]:
result[1, 1] = elapsed
samples[1][1] = sample
Decode MP4 directly from S3¶
Using file-like object input, we can fetch a video stored on AWS S3 and decode it without saving it on local file system.
[22]:
bucket = "pytorch"
key = "torchaudio/tutorial-assets/stream-api/NASAs_Most_Scientifically_Complex_Space_Observatory_Requires_Precision-MP4_small.mp4"
s3_client = boto3.client("s3", config=Config(signature_version=UNSIGNED))
i_sample = 115
Defining Helper class¶
StreamReader supports file-like objects with read
method. In addition to this, if the file-like object has seek
method, StreamReader attempts to use it for more reliable detection of media formats.
However, the seek method of boto3
’s S3 client response object only raises errors to let users know that seek operation is not supported. Therefore we wrap it with a class that does not have seek
method. This way, StreamReader won’t try to use the seek
method.
Note
Due to the nature of streaming, when using file-like object without seek method, some formats are not supported. For example, MP4 formats contain metadata at the beginning of file or at the end. If metadata is located at the end, without seek
method, StreamReader cannot decode streams.
[23]:
# Wrapper to hide the native `seek` method of boto3, which
# only raises an error.
class UnseekableWrapper:
def __init__(self, obj):
self.obj = obj
def read(self, n):
return self.obj.read(n)
def __str__(self):
return str(self.obj)
CPU¶
[24]:
response = s3_client.get_object(Bucket=bucket, Key=key)
src = UnseekableWrapper(response["Body"])
elapsed, sample = test_decode(src, cpu_conf, i_sample)
========================================
* Configuration: {'decoder': 'h264'}
* Source: <botocore.response.StreamingBody object at 0x7fcc9b0b83a0>
========================================
- Chunk: torch.Size([5, 3, 540, 960]) cpu torch.uint8
- Processed 6175 frames.
- Elapsed: 29.465254898000012 seconds.
[25]:
result[2, 0] = elapsed
samples[2][0] = sample
CUDA¶
[26]:
response = s3_client.get_object(Bucket=bucket, Key=key)
src = UnseekableWrapper(response["Body"])
elapsed, sample = test_decode(src, cuda_conf, i_sample)
========================================
* Configuration: {'decoder': 'h264_cuvid', 'hw_accel': 'cuda:0'}
* Source: <botocore.response.StreamingBody object at 0x7fcc9b0b8730>
========================================
- Chunk: torch.Size([5, 3, 540, 960]) cuda:0 torch.uint8
- Processed 6175 frames.
- Elapsed: 3.475249672000018 seconds.
[27]:
result[2, 1] = elapsed
samples[2][1] = sample
Decoding and resizing¶
In the next test, we add preprocessing. NVDEC supports several preprocessing schemes, which are also performed on the chosen hardware. For CPU, we apply the same kind of software preprocessing through FFmpeg’s filter graph.
[28]:
i_sample = 1085
CPU¶
[29]:
cpu_conf = {
"decoder": "h264", # CPU decoding
"filter_desc": "scale=360:240", # Software filter
}
elapsed, sample = test_decode(local_src, cpu_conf, i_sample)
========================================
* Configuration: {'decoder': 'h264', 'filter_desc': 'scale=360:240'}
* Source: input.mp4
========================================
- Chunk: torch.Size([5, 3, 240, 360]) cpu torch.uint8
- Processed 6175 frames.
- Elapsed: 17.26762169899996 seconds.
[30]:
result[3, 0] = elapsed
samples[3][0] = sample
CUDA¶
[31]:
cuda_conf = {
"decoder": "h264_cuvid", # Use CUDA HW decoder
"decoder_option": {
"resize": "360x240", # Then apply HW preprocessing (resize)
},
"hw_accel": "cuda:0", # Then keep the memory on CUDA:0
}
elapsed, sample = test_decode(local_src, cuda_conf, i_sample)
========================================
* Configuration: {'decoder': 'h264_cuvid', 'decoder_option': {'resize': '360x240'}, 'hw_accel': 'cuda:0'}
* Source: input.mp4
========================================
- Chunk: torch.Size([5, 3, 240, 360]) cuda:0 torch.uint8
- Processed 6175 frames.
- Elapsed: 15.233130482000035 seconds.
[32]:
result[3, 1] = elapsed
samples[3][1] = sample
Results¶
The following table summarizes the time it took to decode the same media with CPU and NVDEC. We see significant speedup with NVDEC.
[33]:
res = pd.DataFrame(
result.numpy(),
index=["Decoding (local file)", "Decoding (network file)", "Decoding (file-like object, S3)", "Decoding + Resize"],
columns=["CPU", "NVDEC"],
)
print(res)
CPU NVDEC
Decoding (local file) 43.650681 5.754926
Decoding (network file) 33.747013 15.769150
Decoding (file-like object, S3) 29.465256 3.475250
Decoding + Resize 17.267622 15.233130
The following code shows some frames generated by CPU decoding and NVDEC. They produce seemingly identical results.
[34]:
def yuv_to_rgb(img):
img = img.cpu().to(torch.float)
y = img[..., 0, :, :]
u = img[..., 1, :, :]
v = img[..., 2, :, :]
y /= 255
u = u / 255 - 0.5
v = v / 255 - 0.5
r = y + 1.14 * v
g = y + -0.396 * u - 0.581 * v
b = y + 2.029 * u
rgb = torch.stack([r, g, b], -1)
rgb = (rgb * 255).clamp(0, 255).to(torch.uint8)
return rgb.numpy()
[35]:
f, axs = plt.subplots(4, 2, figsize=[12.8, 19.2])
for i in range(4):
for j in range(2):
axs[i][j].imshow(yuv_to_rgb(samples[i][j]))
axs[i][j].set_title(
f"{'CPU' if j == 0 else 'NVDEC'}{' with resize' if i == 3 else ''}")
plt.plot(block=False)
[35]:
[]
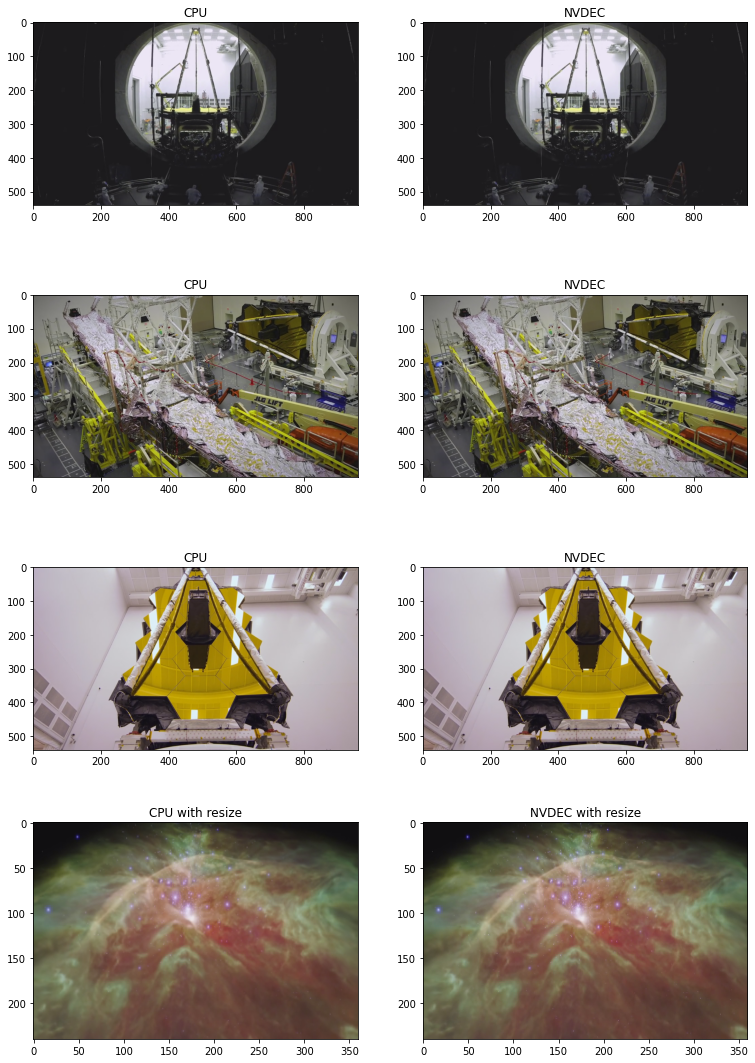
Benchmark NVENC with StreamWriter
¶
Next, we benchmark encoding speed with StreamWriter and NVENC.
[36]:
def test_encode(data, dst, **config):
print("=" * 40)
print("* Configuration:", config)
print("* Destination:", dst)
print("=" * 40)
t0 = time.monotonic()
s = StreamWriter(dst)
s.add_video_stream(**config)
with s.open():
s.write_video_chunk(0, data)
elapsed = time.monotonic() - t0
print()
print(f" - Processed {len(data)} frames.")
print(f" - Elapsed: {elapsed} seconds.")
print()
return elapsed
result = torch.zeros((3, 3))
We use StreamReader
to generate test data.
[37]:
def get_data(frame_rate, height, width, format, duration=15):
src = f"testsrc2=rate={frame_rate}:size={width}x{height}:duration={duration}"
s = StreamReader(src=src, format="lavfi")
s.add_basic_video_stream(-1, format=format)
s.process_all_packets()
video, = s.pop_chunks()
return video
Encode MP4 - 360P¶
For the first test, we compare the time it takes for CPU and NVENC to encode 15 seconds of video with small resolution.
[38]:
pict_config = {
"height": 360,
"width": 640,
"frame_rate": 30000/1001,
"format": "yuv444p",
}
video = get_data(**pict_config)
CPU¶
[39]:
encode_config = {
"encoder": "libx264",
"encoder_format": "yuv444p",
}
result[0, 0] = test_encode(video, "360p_cpu.mp4", **pict_config, **encode_config)
========================================
* Configuration: {'height': 360, 'width': 640, 'frame_rate': 29.97002997002997, 'format': 'yuv444p', 'encoder': 'libx264', 'encoder_format': 'yuv444p'}
* Destination: 360p_cpu.mp4
========================================
- Processed 450 frames.
- Elapsed: 6.311792093000008 seconds.
CUDA (from CPU Tensor)¶
Now we test NVENC. This time, the data is sent from CPU memory to GPU memory as part of encoding.
[40]:
encode_config = {
"encoder": "h264_nvenc", # Use NVENC
"encoder_format": "yuv444p",
"encoder_option": {"gpu": "0"}, # Run encoding on the cuda:0 device
}
result[1, 0] = test_encode(video, "360p_cuda.mp4", **pict_config, **encode_config)
========================================
* Configuration: {'height': 360, 'width': 640, 'frame_rate': 29.97002997002997, 'format': 'yuv444p', 'encoder': 'h264_nvenc', 'encoder_format': 'yuv444p', 'encoder_option': {'gpu': '0'}}
* Destination: 360p_cuda.mp4
========================================
- Processed 450 frames.
- Elapsed: 0.5352325430000064 seconds.
CUDA (from CUDA Tensor)¶
If the data is already present on CUDA, then we can pass it to GPU encoder directly.
[41]:
device = "cuda:0"
encode_config = {
"encoder": "h264_nvenc", # GPU Encoder
"encoder_format": "yuv444p",
"encoder_option": {"gpu": "0"}, # Run encoding on the cuda:0 device
"hw_accel": device, # Data comes from cuda:0 device
}
result[2, 0] = test_encode(video.to(torch.device(device)), "360p_cuda_hw.mp4", **pict_config, **encode_config)
========================================
* Configuration: {'height': 360, 'width': 640, 'frame_rate': 29.97002997002997, 'format': 'yuv444p', 'encoder': 'h264_nvenc', 'encoder_format': 'yuv444p', 'encoder_option': {'gpu': '0'}, 'hw_accel': 'cuda:0'}
* Destination: 360p_cuda_hw.mp4
========================================
- Processed 450 frames.
- Elapsed: 0.7045466739999711 seconds.
Encode MP4 - 720P¶
Let’s run the same tests on video with larger resolution.
[42]:
pict_config = {
"height": 720,
"width": 1280,
"frame_rate": 30000/1001,
"format": "yuv444p",
}
video = get_data(**pict_config)
CPU¶
[43]:
encode_config = {
"encoder": "libx264",
"encoder_format": "yuv444p",
}
result[0, 1] = test_encode(video, "720p_cpu.mp4", **pict_config, **encode_config)
========================================
* Configuration: {'height': 720, 'width': 1280, 'frame_rate': 29.97002997002997, 'format': 'yuv444p', 'encoder': 'libx264', 'encoder_format': 'yuv444p'}
* Destination: 720p_cpu.mp4
========================================
- Processed 450 frames.
- Elapsed: 14.017578084000036 seconds.
CUDA (from CPU Tensor)¶
[44]:
encode_config = {
"encoder": "h264_nvenc",
"encoder_format": "yuv444p",
}
result[1, 1] = test_encode(video, "720p_cuda.mp4", **pict_config, **encode_config)
========================================
* Configuration: {'height': 720, 'width': 1280, 'frame_rate': 29.97002997002997, 'format': 'yuv444p', 'encoder': 'h264_nvenc', 'encoder_format': 'yuv444p'}
* Destination: 720p_cuda.mp4
========================================
- Processed 450 frames.
- Elapsed: 0.9797491379999883 seconds.
CUDA (from CUDA Tensor)¶
[45]:
device = "cuda:0"
encode_config = {
"encoder": "h264_nvenc",
"encoder_format": "yuv444p",
"encoder_option": {"gpu": "0"},
"hw_accel": device,
}
result[2, 1] = test_encode(video.to(torch.device(device)), "720p_cuda_hw.mp4", **pict_config, **encode_config)
========================================
* Configuration: {'height': 720, 'width': 1280, 'frame_rate': 29.97002997002997, 'format': 'yuv444p', 'encoder': 'h264_nvenc', 'encoder_format': 'yuv444p', 'encoder_option': {'gpu': '0'}, 'hw_accel': 'cuda:0'}
* Destination: 720p_cuda_hw.mp4
========================================
- Processed 450 frames.
- Elapsed: 0.7259890020000057 seconds.
Encode MP4 - 1080P¶
We make the video with even larger.
[46]:
pict_config = {
"height": 1080,
"width": 1920,
"frame_rate": 30000/1001,
"format": "yuv444p",
}
video = get_data(**pict_config)
CPU¶
[47]:
encode_config = {
"encoder": "libx264",
"encoder_format": "yuv444p",
}
result[0, 2] = test_encode(video, "1080p_cpu.mp4", **pict_config, **encode_config)
========================================
* Configuration: {'height': 1080, 'width': 1920, 'frame_rate': 29.97002997002997, 'format': 'yuv444p', 'encoder': 'libx264', 'encoder_format': 'yuv444p'}
* Destination: 1080p_cpu.mp4
========================================
- Processed 450 frames.
- Elapsed: 29.441768007999997 seconds.
CUDA (from CPU Tensor)¶
[48]:
encode_config = {
"encoder": "h264_nvenc",
"encoder_format": "yuv444p",
}
result[1, 2] = test_encode(video, "1080p_cuda.mp4", **pict_config, **encode_config)
========================================
* Configuration: {'height': 1080, 'width': 1920, 'frame_rate': 29.97002997002997, 'format': 'yuv444p', 'encoder': 'h264_nvenc', 'encoder_format': 'yuv444p'}
* Destination: 1080p_cuda.mp4
========================================
- Processed 450 frames.
- Elapsed: 1.5768605540000067 seconds.
CUDA (from CUDA Tensor)¶
[49]:
device = "cuda:0"
encode_config = {
"encoder": "h264_nvenc",
"encoder_format": "yuv444p",
"encoder_option": {"gpu": "0"},
"hw_accel": device,
}
result[2, 2] = test_encode(video.to(torch.device(device)), "1080p_cuda_hw.mp4", **pict_config, **encode_config)
========================================
* Configuration: {'height': 1080, 'width': 1920, 'frame_rate': 29.97002997002997, 'format': 'yuv444p', 'encoder': 'h264_nvenc', 'encoder_format': 'yuv444p', 'encoder_option': {'gpu': '0'}, 'hw_accel': 'cuda:0'}
* Destination: 1080p_cuda_hw.mp4
========================================
- Processed 450 frames.
- Elapsed: 1.4002963130000126 seconds.
Result¶
Here is the result.
[50]:
labels = ["CPU", "CUDA (from CPU Tensor)", "CUDA (from CUDA Tensor)"]
columns = ["360P", "720P", "1080P"]
res = pd.DataFrame(
result.numpy(),
index=labels,
columns=columns,
)
print(res)
360P 720P 1080P
CPU 6.311792 14.017578 29.441769
CUDA (from CPU Tensor) 0.535233 0.979749 1.576861
CUDA (from CUDA Tensor) 0.704547 0.725989 1.400296
[51]:
plt.plot(result.T)
plt.legend(labels)
plt.xticks([i for i in range(3)], columns)
plt.grid(visible=True, axis='y')
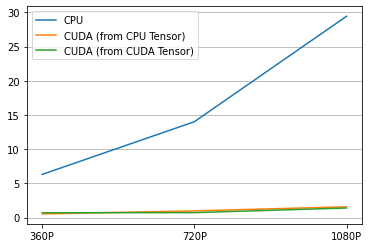
The resulting videos look like the following.
[52]:
from IPython.display import HTML
HTML('''
<div>
<video width=360 controls autoplay>
<source src="https://download.pytorch.org/torchaudio/tutorial-assets/streamwriter_360p_cpu.mp4" type="video/mp4">
</video>
<video width=360 controls autoplay>
<source src="https://download.pytorch.org/torchaudio/tutorial-assets/streamwriter_360p_cuda.mp4" type="video/mp4">
</video>
<video width=360 controls autoplay>
<source src="https://download.pytorch.org/torchaudio/tutorial-assets/streamwriter_360p_cuda_hw.mp4" type="video/mp4">
</video>
</div>
''')
[52]:
Conclusion¶
We looked at how to build FFmpeg libraries with NVDEC/NVENC support and use them from TorchAudio. NVDEC/NVENC provide significant speed up when saving/loading a video.