Note
Go to the end to download the full example code
Transforms v2: End-to-end object detection example¶
Object detection is not supported out of the box by torchvision.transforms
v1, since it only supports images.
torchvision.transforms.v2
enables jointly transforming images, videos, bounding boxes, and masks. This example
showcases an end-to-end object detection training using the stable torchvisio.datasets
and torchvision.models
as
well as the new torchvision.transforms.v2
v2 API.
import pathlib
from collections import defaultdict
import PIL.Image
import torch
import torch.utils.data
import torchvision
def show(sample):
import matplotlib.pyplot as plt
from torchvision.transforms.v2 import functional as F
from torchvision.utils import draw_bounding_boxes
image, target = sample
if isinstance(image, PIL.Image.Image):
image = F.to_image_tensor(image)
image = F.convert_dtype(image, torch.uint8)
annotated_image = draw_bounding_boxes(image, target["boxes"], colors="yellow", width=3)
fig, ax = plt.subplots()
ax.imshow(annotated_image.permute(1, 2, 0).numpy())
ax.set(xticklabels=[], yticklabels=[], xticks=[], yticks=[])
fig.tight_layout()
fig.show()
# We are using BETA APIs, so we deactivate the associated warning, thereby acknowledging that
# some APIs may slightly change in the future
torchvision.disable_beta_transforms_warning()
from torchvision import models, datasets
import torchvision.transforms.v2 as transforms
We start off by loading the CocoDetection
dataset to have a look at what it currently
returns, and we’ll see how to convert it to a format that is compatible with our new transforms.
def load_example_coco_detection_dataset(**kwargs):
# This loads fake data for illustration purposes of this example. In practice, you'll have
# to replace this with the proper data
root = pathlib.Path("assets") / "coco"
return datasets.CocoDetection(str(root / "images"), str(root / "instances.json"), **kwargs)
dataset = load_example_coco_detection_dataset()
sample = dataset[0]
image, target = sample
print(type(image))
print(type(target), type(target[0]), list(target[0].keys()))
loading annotations into memory...
Done (t=0.00s)
creating index...
index created!
<class 'PIL.Image.Image'>
<class 'list'> <class 'dict'> ['segmentation', 'iscrowd', 'image_id', 'bbox', 'category_id', 'id']
The dataset returns a two-tuple with the first item being a PIL.Image.Image
and second one a list of
dictionaries, which each containing the annotations for a single object instance. As is, this format is not compatible
with the torchvision.transforms.v2
, nor with the models. To overcome that, we provide the
wrap_dataset_for_transforms_v2()
function. For
CocoDetection
, this changes the target structure to a single dictionary of lists. It
also adds the key-value-pairs "boxes"
, "masks"
, and "labels"
wrapped in the corresponding
torchvision.datapoints
.
<class 'PIL.Image.Image'>
<class 'dict'> ['segmentation', 'iscrowd', 'image_id', 'bbox', 'category_id', 'id', 'boxes', 'masks', 'labels']
<class 'torchvision.datapoints._bounding_box.BoundingBox'> <class 'torchvision.datapoints._mask.Mask'> <class 'torch.Tensor'>
As baseline, let’s have a look at a sample without transformations:
show(sample)
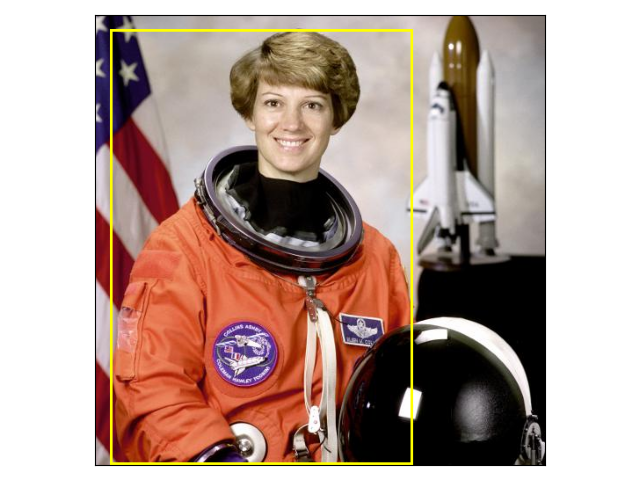
With the dataset properly set up, we can now define the augmentation pipeline. This is done the same way it is done in
torchvision.transforms
v1, but now handles bounding boxes and masks without any extra configuration.
transform = transforms.Compose(
[
transforms.RandomPhotometricDistort(),
transforms.RandomZoomOut(
fill=defaultdict(lambda: 0, {PIL.Image.Image: (123, 117, 104)})
),
transforms.RandomIoUCrop(),
transforms.RandomHorizontalFlip(),
transforms.ToImageTensor(),
transforms.ConvertImageDtype(torch.float32),
transforms.SanitizeBoundingBox(),
]
)
Note
Although the SanitizeBoundingBox
transform is a no-op in this example, but it
should be placed at least once at the end of a detection pipeline to remove degenerate bounding boxes as well as
the corresponding labels and optionally masks. It is particularly critical to add it if
RandomIoUCrop
was used.
Let’s look how the sample looks like with our augmentation pipeline in place:
dataset = load_example_coco_detection_dataset(transforms=transform)
dataset = datasets.wrap_dataset_for_transforms_v2(dataset)
torch.manual_seed(3141)
sample = dataset[0]
show(sample)
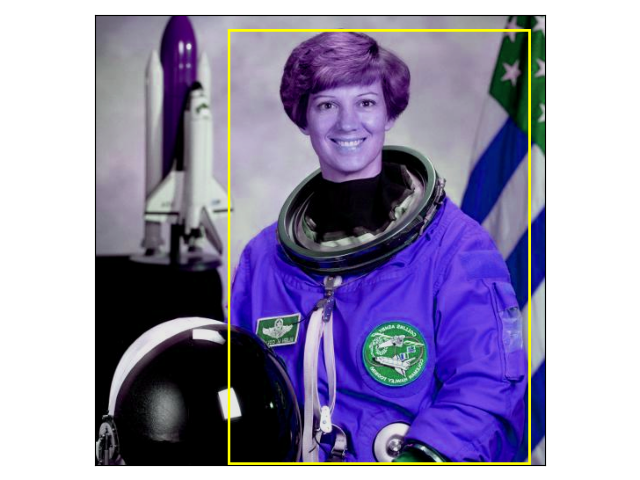
loading annotations into memory...
Done (t=0.00s)
creating index...
index created!
We can see that the color of the image was distorted, we zoomed out on it (off center) and flipped it horizontally. In all of this, the bounding box was transformed accordingly. And without any further ado, we can start training.
data_loader = torch.utils.data.DataLoader(
dataset,
batch_size=2,
# We need a custom collation function here, since the object detection models expect a
# sequence of images and target dictionaries. The default collation function tries to
# `torch.stack` the individual elements, which fails in general for object detection,
# because the number of object instances varies between the samples. This is the same for
# `torchvision.transforms` v1
collate_fn=lambda batch: tuple(zip(*batch)),
)
model = models.get_model("ssd300_vgg16", weights=None, weights_backbone=None).train()
for images, targets in data_loader:
loss_dict = model(images, targets)
print(loss_dict)
# Put your training logic here
break
{'bbox_regression': tensor(4.0019, grad_fn=<DivBackward0>), 'classification': tensor(42.4939, grad_fn=<DivBackward0>)}
Total running time of the script: ( 0 minutes 2.996 seconds)