Hardware-Accelerated Video Decoding and Encoding¶
Author: Moto Hira
This tutorial shows how to use NVIDIA’s hardware video decoder (NVDEC) and encoder (NVENC) with TorchAudio.
Using hardware encoder/decoder improves the speed of loading and saving certain types of videos. Using them in TorchAduio requires additional FFmpeg configuration. This tutorial goes over how to compile FFmpeg, and compare the speed it takes to process video.
WARNING
This tutorial instsalls FFmpeg in system directory. If you run this tutorial on your system, please adjust the build configuration accordingly.
NOTE
This tutorial was authored in Google Colab, and is tailored to Google Colab’s specifications. Please check out this tutorial in Google Colab.
To use NVENC/NVDEC with TorchAudio, the following items are required.
NVIDIA GPU with hardware video decoder/encoder.
FFmpeg libraries compiled with NVDEC/NVENC support. †
PyTorch / TorchAudio with CUDA support.
TorchAudio’s official binary distributions are compiled with FFmpeg 4 libraries, and they contain the logic required for hardware-based decoding/encoding.
In the following sections, we build FFmpeg 4 libraries with NVDEC/NVENC support, then we demonstrate the performance imrovement using TorchAudio’s StreamReader
/StreamWriter
.
† For details on NVDEC/NVENC and FFmpeg, please refer to the following articles.
https://docs.nvidia.com/video-technologies/video-codec-sdk/nvdec-video-decoder-api-prog-guide/
https://docs.nvidia.com/video-technologies/video-codec-sdk/ffmpeg-with-nvidia-gpu/#compiling-ffmpeg
https://developer.nvidia.com/blog/nvidia-ffmpeg-transcoding-guide/
Check the available GPU¶
[1]:
!nvidia-smi
Fri Oct 7 13:01:26 2022
+-----------------------------------------------------------------------------+
| NVIDIA-SMI 460.32.03 Driver Version: 460.32.03 CUDA Version: 11.2 |
|-------------------------------+----------------------+----------------------+
| GPU Name Persistence-M| Bus-Id Disp.A | Volatile Uncorr. ECC |
| Fan Temp Perf Pwr:Usage/Cap| Memory-Usage | GPU-Util Compute M. |
| | | MIG M. |
|===============================+======================+======================|
| 0 Tesla T4 Off | 00000000:00:04.0 Off | 0 |
| N/A 56C P8 10W / 70W | 0MiB / 15109MiB | 0% Default |
| | | N/A |
+-------------------------------+----------------------+----------------------+
+-----------------------------------------------------------------------------+
| Processes: |
| GPU GI CI PID Type Process name GPU Memory |
| ID ID Usage |
|=============================================================================|
| No running processes found |
+-----------------------------------------------------------------------------+
Update PyTorch and TorchAudio with nightly builds¶
Until TorchAudio 0.13 is released, we need to use the nightly builds of PyTorch and TorchAudio.
[2]:
!pip uninstall -y -q torch torchaudio torchvision torchtext
!pip install --progress-bar off --pre torch torchaudio --extra-index-url https://download.pytorch.org/whl/nightly/cu116 2> /dev/null
Looking in indexes: https://pypi.org/simple, https://us-python.pkg.dev/colab-wheels/public/simple/, https://download.pytorch.org/whl/nightly/cu116
Collecting torch
Downloading https://download.pytorch.org/whl/nightly/cu116/torch-1.14.0.dev20221007%2Bcu116-cp37-cp37m-linux_x86_64.whl (2286.1 MB)
Collecting torchaudio
Downloading https://download.pytorch.org/whl/nightly/cu116/torchaudio-0.13.0.dev20221006%2Bcu116-cp37-cp37m-linux_x86_64.whl (4.2 MB)
Requirement already satisfied: typing-extensions in /usr/local/lib/python3.7/dist-packages (from torch) (4.1.1)
Collecting torch
Downloading https://download.pytorch.org/whl/nightly/cu116/torch-1.13.0.dev20221006%2Bcu116-cp37-cp37m-linux_x86_64.whl (1983.0 MB)
Installing collected packages: torch, torchaudio
Successfully installed torch-1.13.0.dev20221006+cu116 torchaudio-0.13.0.dev20221006+cu116
Build FFmpeg libraries with Nvidia NVDEC/NVENC support¶
Install NVIDIA Video Codec Headers¶
To build FFmpeg with NVDEC/NVENC, we first install the headers that FFmpeg uses to interact with Video Codec SDK.
[3]:
!git clone https://git.videolan.org/git/ffmpeg/nv-codec-headers.git
# Note: Google Colab's GPU has NVENC API ver 11.0, so we checkout 11.0 tag.
!cd nv-codec-headers && git checkout n11.0.10.1 && sudo make install
Cloning into 'nv-codec-headers'...
remote: Enumerating objects: 819, done.
remote: Counting objects: 100% (819/819), done.
remote: Compressing objects: 100% (697/697), done.
remote: Total 819 (delta 439), reused 0 (delta 0)
Receiving objects: 100% (819/819), 156.42 KiB | 410.00 KiB/s, done.
Resolving deltas: 100% (439/439), done.
Note: checking out 'n11.0.10.1'.
You are in 'detached HEAD' state. You can look around, make experimental
changes and commit them, and you can discard any commits you make in this
state without impacting any branches by performing another checkout.
If you want to create a new branch to retain commits you create, you may
do so (now or later) by using -b with the checkout command again. Example:
git checkout -b <new-branch-name>
HEAD is now at 315ad74 add cuMemcpy
sed 's#@@PREFIX@@#/usr/local#' ffnvcodec.pc.in > ffnvcodec.pc
install -m 0755 -d '/usr/local/include/ffnvcodec'
install -m 0644 include/ffnvcodec/*.h '/usr/local/include/ffnvcodec'
install -m 0755 -d '/usr/local/lib/pkgconfig'
install -m 0644 ffnvcodec.pc '/usr/local/lib/pkgconfig'
Download FFmpeg source code¶
Next we download the source code of FFmpeg 4. Any version later than 4.1 should work. We use 4.4.2 here.
[4]:
!wget -q https://github.com/FFmpeg/FFmpeg/archive/refs/tags/n4.4.2.tar.gz
!tar -xf n4.4.2.tar.gz
!mv FFmpeg-n4.4.2 ffmpeg
Install FFmpeg build and runtime dependencies¶
In the later test, we use H264 video codec and HTTPS protocol, so we install the libraries for them here.
[5]:
!apt -qq update
!apt -qq install -y yasm libx264-dev libgnutls28-dev
STRIP install-libavutil-shared
... Omitted for brevity ...
Setting up libx264-dev:amd64 (2:0.152.2854+gite9a5903-2) ...
Setting up yasm (1.3.0-2build1) ...
Setting up libunbound2:amd64 (1.6.7-1ubuntu2.5) ...
Setting up libp11-kit-dev:amd64 (0.23.9-2ubuntu0.1) ...
Setting up libtasn1-6-dev:amd64 (4.13-2) ...
Setting up libtasn1-doc (4.13-2) ...
Setting up libgnutlsxx28:amd64 (3.5.18-1ubuntu1.6) ...
Setting up libgnutls-dane0:amd64 (3.5.18-1ubuntu1.6) ...
Setting up libgnutls-openssl27:amd64 (3.5.18-1ubuntu1.6) ...
Setting up libgmpxx4ldbl:amd64 (2:6.1.2+dfsg-2) ...
Setting up libidn2-dev:amd64 (2.0.4-1.1ubuntu0.2) ...
Setting up libidn2-0-dev (2.0.4-1.1ubuntu0.2) ...
Setting up libgmp-dev:amd64 (2:6.1.2+dfsg-2) ...
Setting up nettle-dev:amd64 (3.4.1-0ubuntu0.18.04.1) ...
Setting up libgnutls28-dev:amd64 (3.5.18-1ubuntu1.6) ...
Processing triggers for man-db (2.8.3-2ubuntu0.1) ...
Processing triggers for libc-bin (2.27-3ubuntu1.6) ...
Configure FFmpeg build with Nvidia CUDA hardware support¶
Next we configure FFmpeg build. Note the following:
We provide flags like
-I/usr/local/cuda/include
,-L/usr/local/cuda/lib64
to let the build process know where the CUDA libraries are found.We provide flags like
--enable-nvdec
and--enable-nvenc
to enable NVDEC/NVENC. Please check out the Transcoding Guide† for the detail.We also provide NVCC flags with compute capability 37. This is because by default the configuration script verifies NVCC by compiling sample code targeting compute capability 30, which is too old for CUDA 11.
Many features are disabled to reduce the compilation time.
We install the library in
/usr/lib/
, which is one of the active search path of the dynamic loader. Doing so allows the resulting libraries to be found without requiring a restart of the current session. This might be an undesirable location, e.g. when one isn’t using a disposable VM.
† NVIDIA FFmpeg Transcoding Guide https://developer.nvidia.com/blog/nvidia-ffmpeg-transcoding-guide/
[6]:
# NOTE:
# When the configure script of FFmpeg 4 checks nvcc, it uses compute
# capability of 30 (3.0) by default. CUDA 11, however, does not support
# compute capability 30.
# Here, we use 37, which is supported by CUDA 11 and both K80 and T4.
#
# Tesla K80: 37
# NVIDIA T4: 75
%env ccap=37
# NOTE:
# We disable most of the features to speed up compilation
# The necessary components are
# - demuxer: mov, aac
# - decoder: h264, h264_nvdec
# - muxer: mp4
# - encoder: libx264, h264_nvenc
# - gnutls (HTTPS)
#
# Additionally, we use FFmpeg's virtual input device to generate
# test video data. This requires
# - input device: lavfi
# - filter: testsrc2
# - decoder: rawvideo
#
!cd ffmpeg && ./configure \
--prefix='/usr/' \
--extra-cflags='-I/usr/local/cuda/include' \
--extra-ldflags='-L/usr/local/cuda/lib64' \
--nvccflags="-gencode arch=compute_${ccap},code=sm_${ccap} -O2" \
--disable-doc \
--disable-static \
--disable-bsfs \
--disable-decoders \
--disable-encoders \
--disable-filters \
--disable-demuxers \
--disable-devices \
--disable-muxers \
--disable-parsers \
--disable-postproc \
--disable-protocols \
--enable-decoder=aac \
--enable-decoder=h264 \
--enable-decoder=h264_cuvid \
--enable-decoder=rawvideo \
--enable-indev=lavfi \
--enable-encoder=libx264 \
--enable-encoder=h264_nvenc \
--enable-demuxer=mov \
--enable-muxer=mp4 \
--enable-filter=scale \
--enable-filter=testsrc2 \
--enable-protocol=file \
--enable-protocol=https \
--enable-gnutls \
--enable-shared \
--enable-gpl \
--enable-nonfree \
--enable-cuda-nvcc \
--enable-libx264 \
--enable-nvenc \
--enable-cuvid \
--enable-nvdec
env: ccap=37
install prefix /usr/
source path .
C compiler gcc
C library glibc
ARCH x86 (generic)
big-endian no
runtime cpu detection yes
standalone assembly yes
x86 assembler yasm
MMX enabled yes
MMXEXT enabled yes
3DNow! enabled yes
3DNow! extended enabled yes
SSE enabled yes
SSSE3 enabled yes
AESNI enabled yes
AVX enabled yes
AVX2 enabled yes
AVX-512 enabled yes
XOP enabled yes
FMA3 enabled yes
FMA4 enabled yes
i686 features enabled yes
CMOV is fast yes
EBX available yes
EBP available yes
debug symbols yes
strip symbols yes
optimize for size no
optimizations yes
static no
shared yes
postprocessing support no
network support yes
threading support pthreads
safe bitstream reader yes
texi2html enabled no
perl enabled yes
pod2man enabled yes
makeinfo enabled no
makeinfo supports HTML no
External libraries:
alsa libx264 lzma
bzlib libxcb zlib
gnutls libxcb_shape
iconv libxcb_xfixes
External libraries providing hardware acceleration:
cuda cuvid nvenc
cuda_llvm ffnvcodec v4l2_m2m
cuda_nvcc nvdec
Libraries:
avcodec avformat swscale
avdevice avutil
avfilter swresample
Programs:
ffmpeg ffprobe
Enabled decoders:
aac hevc rawvideo
av1 mjpeg vc1
h263 mpeg1video vp8
h264 mpeg2video vp9
h264_cuvid mpeg4
Enabled encoders:
h264_nvenc libx264
Enabled hwaccels:
av1_nvdec mpeg1_nvdec vp8_nvdec
h264_nvdec mpeg2_nvdec vp9_nvdec
hevc_nvdec mpeg4_nvdec wmv3_nvdec
mjpeg_nvdec vc1_nvdec
Enabled parsers:
h263 mpeg4video vp9
Enabled demuxers:
mov
Enabled muxers:
mov mp4
Enabled protocols:
file tcp
https tls
Enabled filters:
aformat hflip transpose
anull null trim
atrim scale vflip
format testsrc2
Enabled bsfs:
aac_adtstoasc null vp9_superframe_split
h264_mp4toannexb vp9_superframe
Enabled indevs:
lavfi
Enabled outdevs:
License: nonfree and unredistributable
Build and install FFmpeg¶
[7]:
!cd ffmpeg && make clean && make -j > /dev/null 2>&1
!cd ffmpeg && make install
INSTALL libavdevice/libavdevice.so
INSTALL libavfilter/libavfilter.so
INSTALL libavformat/libavformat.so
INSTALL libavcodec/libavcodec.so
INSTALL libswresample/libswresample.so
INSTALL libswscale/libswscale.so
INSTALL libavutil/libavutil.so
INSTALL install-progs-yes
INSTALL ffmpeg
INSTALL ffprobe
Check FFmpeg installation¶
Let’s do a quick sanity check to confirm that the FFmpeg we built works.
[8]:
!ffprobe -hide_banner -decoders | grep h264
VFS..D h264 H.264 / AVC / MPEG-4 AVC / MPEG-4 part 10
V..... h264_cuvid Nvidia CUVID H264 decoder (codec h264)
[9]:
!ffmpeg -hide_banner -encoders | grep 264
V..... libx264 libx264 H.264 / AVC / MPEG-4 AVC / MPEG-4 part 10 (codec h264)
V....D h264_nvenc NVIDIA NVENC H.264 encoder (codec h264)
The following command fetches video from remote server, decode with NVDEC (cuvid) and re-encode with NVENC. If this command does not work, then there is an issue with FFmpeg installation, and TorchAudio would not be able to use them either.
[10]:
!ffmpeg -hide_banner -y -vsync 0 -hwaccel cuvid -hwaccel_output_format cuda -c:v h264_cuvid -resize 360x240 -i "https://download.pytorch.org/torchaudio/tutorial-assets/stream-api/NASAs_Most_Scientifically_Complex_Space_Observatory_Requires_Precision-MP4_small.mp4" -c:a copy -c:v h264_nvenc -b:v 5M test.mp4
Input #0, mov,mp4,m4a,3gp,3g2,mj2, from 'https://download.pytorch.org/torchaudio/tutorial-assets/stream-api/NASAs_Most_Scientifically_Complex_Space_Observatory_Requires_Precision-MP4_small.mp4':
Metadata:
major_brand : mp42
minor_version : 512
compatible_brands: mp42iso2avc1mp41
encoder : Lavf58.76.100
Duration: 00:03:26.04, start: 0.000000, bitrate: 1294 kb/s
Stream #0:0(eng): Video: h264 (High) (avc1 / 0x31637661), yuv420p(tv, bt709), 960x540 [SAR 1:1 DAR 16:9], 1156 kb/s, 29.97 fps, 29.97 tbr, 30k tbn, 59.94 tbc (default)
Metadata:
handler_name : ?Mainconcept Video Media Handler
vendor_id : [0][0][0][0]
Stream #0:1(eng): Audio: aac (LC) (mp4a / 0x6134706D), 48000 Hz, stereo, fltp, 128 kb/s (default)
Metadata:
handler_name : #Mainconcept MP4 Sound Media Handler
vendor_id : [0][0][0][0]
Stream mapping:
Stream #0:0 -> #0:0 (h264 (h264_cuvid) -> h264 (h264_nvenc))
Stream #0:1 -> #0:1 (copy)
Press [q] to stop, [?] for help
Output #0, mp4, to 'test.mp4':
Metadata:
major_brand : mp42
minor_version : 512
compatible_brands: mp42iso2avc1mp41
encoder : Lavf58.76.100
Stream #0:0(eng): Video: h264 (Main) (avc1 / 0x31637661), cuda(tv, bt709, progressive), 360x240 [SAR 1:1 DAR 3:2], q=2-31, 5000 kb/s, 29.97 fps, 30k tbn (default)
Metadata:
handler_name : ?Mainconcept Video Media Handler
vendor_id : [0][0][0][0]
encoder : Lavc58.134.100 h264_nvenc
Side data:
cpb: bitrate max/min/avg: 0/0/5000000 buffer size: 10000000 vbv_delay: N/A
Stream #0:1(eng): Audio: aac (LC) (mp4a / 0x6134706D), 48000 Hz, stereo, fltp, 128 kb/s (default)
Metadata:
handler_name : #Mainconcept MP4 Sound Media Handler
vendor_id : [0][0][0][0]
frame= 6175 fps=1712 q=11.0 Lsize= 37935kB time=00:03:26.01 bitrate=1508.5kbits/s speed=57.1x
video:34502kB audio:3234kB subtitle:0kB other streams:0kB global headers:0kB muxing overhead: 0.526932%
Benchmarking GPU Encoding and Decoding¶
Now that FFmpeg and the resulting libraries are ready to use, we test NVDEC/NVENC with TorchAudio. For the basics of TorchAudio’s streaming APIs, please refer to Media I/O tutorials.
Note
If you rebuild FFmpeg after importing torchaudio, you’ll need to restart the session to activate the newly built FFmpeg libraries.
[11]:
import torch
import torchaudio
print(torch.__version__)
print(torchaudio.__version__)
from torchaudio.io import StreamReader, StreamWriter
1.13.0.dev20221006+cu116
0.13.0.dev20221006+cu116
[12]:
import time
import matplotlib.pyplot as plt
import pandas as pd
pd.set_option('display.max_rows', None)
pd.set_option('display.max_columns', None)
Benchmark NVDEC with StreamReader
¶
First we test hardware decoding, and we fetch video from multiple locations (local file, network file, AWS S3) and use NVDEC to decod them.
[13]:
!pip3 install --progress-bar off boto3 2> /dev/null
Looking in indexes: https://pypi.org/simple, https://us-python.pkg.dev/colab-wheels/public/simple/
Collecting boto3
Downloading boto3-1.24.88-py3-none-any.whl (132 kB)
Collecting s3transfer<0.7.0,>=0.6.0
Downloading s3transfer-0.6.0-py3-none-any.whl (79 kB)
Collecting jmespath<2.0.0,>=0.7.1
Downloading jmespath-1.0.1-py3-none-any.whl (20 kB)
Collecting botocore<1.28.0,>=1.27.88
Downloading botocore-1.27.88-py3-none-any.whl (9.2 MB)
Collecting urllib3<1.27,>=1.25.4
Downloading urllib3-1.26.12-py2.py3-none-any.whl (140 kB)
Requirement already satisfied: python-dateutil<3.0.0,>=2.1 in /usr/local/lib/python3.7/dist-packages (from botocore<1.28.0,>=1.27.88->boto3) (2.8.2)
Requirement already satisfied: six>=1.5 in /usr/local/lib/python3.7/dist-packages (from python-dateutil<3.0.0,>=2.1->botocore<1.28.0,>=1.27.88->boto3) (1.15.0)
Installing collected packages: urllib3, jmespath, botocore, s3transfer, boto3
Attempting uninstall: urllib3
Found existing installation: urllib3 1.24.3
Uninstalling urllib3-1.24.3:
Successfully uninstalled urllib3-1.24.3
Successfully installed boto3-1.24.88 botocore-1.27.88 jmespath-1.0.1 s3transfer-0.6.0 urllib3-1.26.12
[14]:
import boto3
from botocore import UNSIGNED
from botocore.config import Config
print(boto3.__version__)
1.24.88
[15]:
!wget -q -O input.mp4 "https://download.pytorch.org/torchaudio/tutorial-assets/stream-api/NASAs_Most_Scientifically_Complex_Space_Observatory_Requires_Precision-MP4_small.mp4"
First, we define the functions we’ll use for testing.
Funcion test_decode
decodes the given source from start to end, and it reports the elapsed time, and returns one image frmae as a sample.
[16]:
result = torch.zeros((4, 2))
samples = [[None, None] for _ in range(4)]
def test_decode(src, config, i_sample):
print("=" * 40)
print("* Configuration:", config)
print("* Source:", src)
print("=" * 40)
s = StreamReader(src)
s.add_video_stream(5, **config)
t0 = time.monotonic()
num_frames = 0
for i, (chunk, ) in enumerate(s.stream()):
if i == 0:
print(' - Chunk:', chunk.shape, chunk.device, chunk.dtype)
if i == i_sample:
sample = chunk[0]
num_frames += chunk.shape[0]
elapsed = time.monotonic() - t0
print()
print(f" - Processed {num_frames} frames.")
print(f" - Elapsed: {elapsed} seconds.")
print()
return elapsed, sample
Decode MP4 from local file¶
For the first test, we compare the time it takes for CPU and NVDEC to decode 250MB of MP4 video.
[17]:
local_src = "input.mp4"
i_sample = 520
CPU¶
[18]:
cpu_conf = {
"decoder": "h264", # CPU decoding
}
elapsed, sample = test_decode(local_src, cpu_conf, i_sample)
========================================
* Configuration: {'decoder': 'h264'}
* Source: input.mp4
========================================
- Chunk: torch.Size([5, 3, 540, 960]) cpu torch.uint8
- Processed 6175 frames.
- Elapsed: 46.691246449000005 seconds.
[19]:
result[0, 0] = elapsed
samples[0][0] = sample
CUDA¶
[20]:
cuda_conf = {
"decoder": "h264_cuvid", # Use CUDA HW decoder
"hw_accel": "cuda:0", # Then keep the memory on CUDA:0
}
elapsed, sample = test_decode(local_src, cuda_conf, i_sample)
========================================
* Configuration: {'decoder': 'h264_cuvid', 'hw_accel': 'cuda:0'}
* Source: input.mp4
========================================
- Chunk: torch.Size([5, 3, 540, 960]) cuda:0 torch.uint8
- Processed 6175 frames.
- Elapsed: 6.117441406000012 seconds.
[21]:
result[0, 1] = elapsed
samples[0][1] = sample
Decode MP4 from network¶
Let’s run the same test on the source retrieved via network on-the-fly.
[22]:
network_src = "https://download.pytorch.org/torchaudio/tutorial-assets/stream-api/NASAs_Most_Scientifically_Complex_Space_Observatory_Requires_Precision-MP4_small.mp4"
i_sample = 750
CPU¶
[23]:
elapsed, sample = test_decode(network_src, cpu_conf, i_sample)
========================================
* Configuration: {'decoder': 'h264'}
* Source: https://download.pytorch.org/torchaudio/tutorial-assets/stream-api/NASAs_Most_Scientifically_Complex_Space_Observatory_Requires_Precision-MP4_small.mp4
========================================
- Chunk: torch.Size([5, 3, 540, 960]) cpu torch.uint8
- Processed 6175 frames.
- Elapsed: 46.460909987000036 seconds.
[24]:
result[1, 0] = elapsed
samples[1][0] = sample
CUDA¶
[25]:
elapsed, sample = test_decode(network_src, cuda_conf, i_sample)
========================================
* Configuration: {'decoder': 'h264_cuvid', 'hw_accel': 'cuda:0'}
* Source: https://download.pytorch.org/torchaudio/tutorial-assets/stream-api/NASAs_Most_Scientifically_Complex_Space_Observatory_Requires_Precision-MP4_small.mp4
========================================
- Chunk: torch.Size([5, 3, 540, 960]) cuda:0 torch.uint8
- Processed 6175 frames.
- Elapsed: 4.23164078800005 seconds.
[26]:
result[1, 1] = elapsed
samples[1][1] = sample
Decode MP4 directly from S3¶
Using file-like object input, we can fetch a video stored on AWS S3 and decode it without saving it on local file system.
[27]:
bucket = "pytorch"
key = "torchaudio/tutorial-assets/stream-api/NASAs_Most_Scientifically_Complex_Space_Observatory_Requires_Precision-MP4_small.mp4"
s3_client = boto3.client("s3", config=Config(signature_version=UNSIGNED))
i_sample = 115
Defining Helper class¶
StreamReader supports file-like objects with read
method. In addition to this, if the file-like object has seek
method, StreamReader attempts to use it for more reliable detection of media formats.
However, the seek method of boto3
’s S3 client response object only raises errors to let users know that seek operation is not supported. Therefore we wrap it with a class that does not have seek
method. This way, StreamReader won’t try to use the seek
method.
Note
Due to the nature of streaming, when using file-like object without seek method, some formats are not supported. For example, MP4 formats contain metadata at the beginning of file or at the end. If metadata is located at the end, without seek
method, StreamReader cannot decode streams.
[28]:
# Wrapper to hide the native `seek` method of boto3, which
# only raises an error.
class UnseekableWrapper:
def __init__(self, obj):
self.obj = obj
def read(self, n):
return self.obj.read(n)
def __str__(self):
return str(self.obj)
CPU¶
[29]:
response = s3_client.get_object(Bucket=bucket, Key=key)
src = UnseekableWrapper(response["Body"])
elapsed, sample = test_decode(src, cpu_conf, i_sample)
========================================
* Configuration: {'decoder': 'h264'}
* Source: <botocore.response.StreamingBody object at 0x7fb991dddfd0>
========================================
- Chunk: torch.Size([5, 3, 540, 960]) cpu torch.uint8
- Processed 6175 frames.
- Elapsed: 40.758733775999985 seconds.
[30]:
result[2, 0] = elapsed
samples[2][0] = sample
CUDA¶
[31]:
response = s3_client.get_object(Bucket=bucket, Key=key)
src = UnseekableWrapper(response["Body"])
elapsed, sample = test_decode(src, cuda_conf, i_sample)
========================================
* Configuration: {'decoder': 'h264_cuvid', 'hw_accel': 'cuda:0'}
* Source: <botocore.response.StreamingBody object at 0x7fb991d390d0>
========================================
- Chunk: torch.Size([5, 3, 540, 960]) cuda:0 torch.uint8
- Processed 6175 frames.
- Elapsed: 4.620101478000038 seconds.
[32]:
result[2, 1] = elapsed
samples[2][1] = sample
Decoding and resizing¶
In the next test, we add preprocessing. NVDEC supports several preprocessing schemes, which are also performed on the chosen hardware. For CPU, we apply the same kind of software preprocessing through FFmpeg’s filter graph.
[33]:
i_sample = 1085
CPU¶
[34]:
cpu_conf = {
"decoder": "h264", # CPU decoding
"filter_desc": "scale=360:240", # Software filter
}
elapsed, sample = test_decode(local_src, cpu_conf, i_sample)
========================================
* Configuration: {'decoder': 'h264', 'filter_desc': 'scale=360:240'}
* Source: input.mp4
========================================
- Chunk: torch.Size([5, 3, 240, 360]) cpu torch.uint8
- Processed 6175 frames.
- Elapsed: 19.082725973000038 seconds.
[35]:
result[3, 0] = elapsed
samples[3][0] = sample
CUDA¶
[36]:
cuda_conf = {
"decoder": "h264_cuvid", # Use CUDA HW decoder
"decoder_option": {
"resize": "360x240", # Then apply HW preprocessing (resize)
},
"hw_accel": "cuda:0", # Then keep the memory on CUDA:0
}
elapsed, sample = test_decode(local_src, cuda_conf, i_sample)
========================================
* Configuration: {'decoder': 'h264_cuvid', 'decoder_option': {'resize': '360x240'}, 'hw_accel': 'cuda:0'}
* Source: input.mp4
========================================
- Chunk: torch.Size([5, 3, 240, 360]) cuda:0 torch.uint8
- Processed 6175 frames.
- Elapsed: 4.157032522999998 seconds.
[37]:
result[3, 1] = elapsed
samples[3][1] = sample
Results¶
The following table summarizes the time it took to decode the same media with CPU and NVDEC. We see significant speedup with NVDEC.
[38]:
res = pd.DataFrame(
result.numpy(),
index=["Decoding (local file)", "Decoding (network file)", "Decoding (file-like object, S3)", "Decoding + Resize"],
columns=["CPU", "NVDEC"],
)
print(res)
CPU NVDEC
Decoding (local file) 46.691246 6.117441
Decoding (network file) 46.460911 4.231641
Decoding (file-like object, S3) 40.758736 4.620101
Decoding + Resize 19.082726 4.157032
The following code shows some frames generated by CPU decoding and NVDEC. They produce seemingly identical results.
[39]:
def yuv_to_rgb(img):
img = img.cpu().to(torch.float)
y = img[..., 0, :, :]
u = img[..., 1, :, :]
v = img[..., 2, :, :]
y /= 255
u = u / 255 - 0.5
v = v / 255 - 0.5
r = y + 1.14 * v
g = y + -0.396 * u - 0.581 * v
b = y + 2.029 * u
rgb = torch.stack([r, g, b], -1)
rgb = (rgb * 255).clamp(0, 255).to(torch.uint8)
return rgb.numpy()
[40]:
f, axs = plt.subplots(4, 2, figsize=[12.8, 19.2])
for i in range(4):
for j in range(2):
axs[i][j].imshow(yuv_to_rgb(samples[i][j]))
axs[i][j].set_title(
f"{'CPU' if j == 0 else 'NVDEC'}{' with resize' if i == 3 else ''}")
plt.plot(block=False)
[40]:
[]
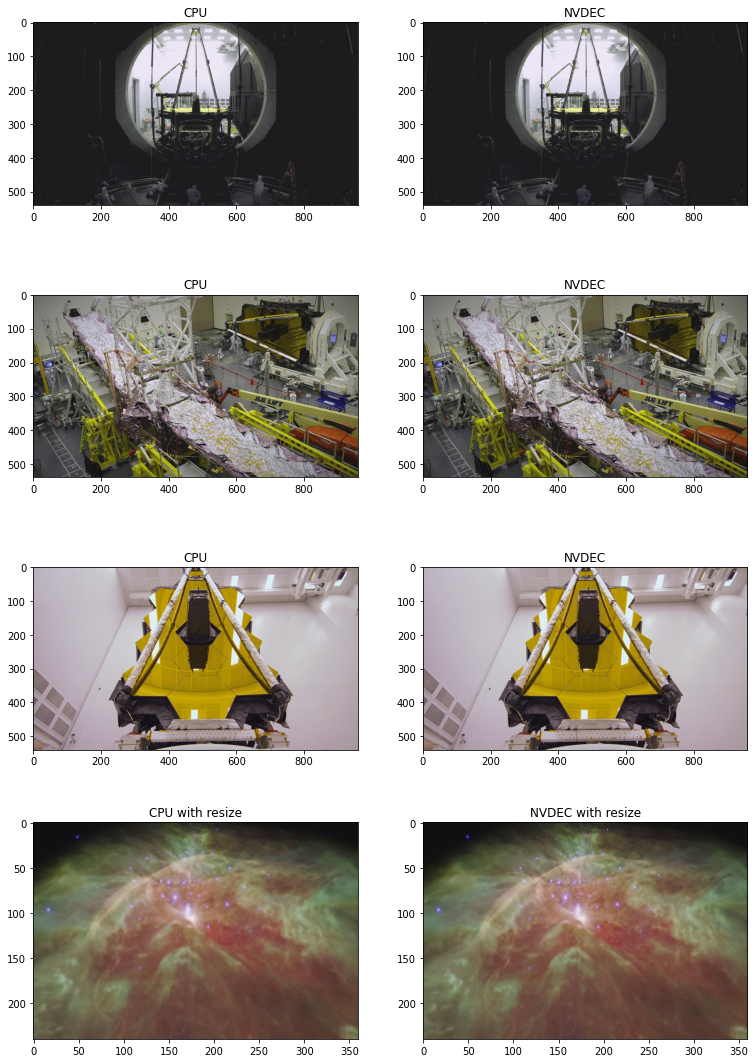
Benchmark NVENC with StreamWriter
¶
Next, we benchmark encoding speed with StreamWriter and NVENC.
[41]:
def test_encode(data, dst, **config):
print("=" * 40)
print("* Configuration:", config)
print("* Destination:", dst)
print("=" * 40)
s = StreamWriter(dst)
s.add_video_stream(**config)
t0 = time.monotonic()
with s.open():
s.write_video_chunk(0, data)
elapsed = time.monotonic() - t0
print()
print(f" - Processed {len(data)} frames.")
print(f" - Elapsed: {elapsed} seconds.")
print()
return elapsed
result = torch.zeros((3, 3))
We use StreamReader
to generate test data.
[42]:
def get_data(frame_rate, height, width, format, duration=15):
src = f"testsrc2=rate={frame_rate}:size={width}x{height}:duration={duration}"
s = StreamReader(src=src, format="lavfi")
s.add_basic_video_stream(-1, format=format)
s.process_all_packets()
video, = s.pop_chunks()
return video
Encode MP4 - 360P¶
For the first test, we compare the time it takes for CPU and NVENC to encode 15 seconds of video with small resolution.
[43]:
pict_config = {
"height": 360,
"width": 640,
"frame_rate": 30000/1001,
"format": "yuv444p",
}
video = get_data(**pict_config)
CPU¶
[44]:
encode_config = {
"encoder": "libx264",
"encoder_format": "yuv444p",
}
result[0, 0] = test_encode(video, "360p_cpu.mp4", **pict_config, **encode_config)
========================================
* Configuration: {'height': 360, 'width': 640, 'frame_rate': 29.97002997002997, 'format': 'yuv444p', 'encoder': 'libx264', 'encoder_format': 'yuv444p'}
* Destination: 360p_cpu.mp4
========================================
- Processed 450 frames.
- Elapsed: 3.280829835000077 seconds.
CUDA (from CPU Tensor)¶
Now we test NVENC. This time, the data is sent from CPU memory to GPU memory as part of encoding.
[45]:
encode_config = {
"encoder": "h264_nvenc", # Use NVENC
"encoder_format": "yuv444p",
"encoder_option": {"gpu": "0"}, # Run encoding on the cuda:0 device
}
result[1, 0] = test_encode(video, "360p_cuda.mp4", **pict_config, **encode_config)
========================================
* Configuration: {'height': 360, 'width': 640, 'frame_rate': 29.97002997002997, 'format': 'yuv444p', 'encoder': 'h264_nvenc', 'encoder_format': 'yuv444p', 'encoder_option': {'gpu': '0'}}
* Destination: 360p_cuda.mp4
========================================
- Processed 450 frames.
- Elapsed: 0.34294435300000714 seconds.
CUDA (from CUDA Tensor)¶
If the data is already present on CUDA, then we can pass it to GPU encoder directly.
[46]:
device = "cuda:0"
encode_config = {
"encoder": "h264_nvenc", # GPU Encoder
"encoder_format": "yuv444p",
"encoder_option": {"gpu": "0"}, # Run encoding on the cuda:0 device
"hw_accel": device, # Data comes from cuda:0 device
}
result[2, 0] = test_encode(video.to(torch.device(device)), "360p_cuda_hw.mp4", **pict_config, **encode_config)
========================================
* Configuration: {'height': 360, 'width': 640, 'frame_rate': 29.97002997002997, 'format': 'yuv444p', 'encoder': 'h264_nvenc', 'encoder_format': 'yuv444p', 'encoder_option': {'gpu': '0'}, 'hw_accel': 'cuda:0'}
* Destination: 360p_cuda_hw.mp4
========================================
- Processed 450 frames.
- Elapsed: 0.2424524550000342 seconds.
Encode MP4 - 720P¶
Let’s run the same tests on video with larger resolution.
[47]:
pict_config = {
"height": 720,
"width": 1280,
"frame_rate": 30000/1001,
"format": "yuv444p",
}
video = get_data(**pict_config)
CPU¶
[48]:
encode_config = {
"encoder": "libx264",
"encoder_format": "yuv444p",
}
result[0, 1] = test_encode(video, "720p_cpu.mp4", **pict_config, **encode_config)
========================================
* Configuration: {'height': 720, 'width': 1280, 'frame_rate': 29.97002997002997, 'format': 'yuv444p', 'encoder': 'libx264', 'encoder_format': 'yuv444p'}
* Destination: 720p_cpu.mp4
========================================
- Processed 450 frames.
- Elapsed: 11.638768525999922 seconds.
CUDA (from CPU Tensor)¶
[49]:
encode_config = {
"encoder": "h264_nvenc",
"encoder_format": "yuv444p",
}
result[1, 1] = test_encode(video, "720p_cuda.mp4", **pict_config, **encode_config)
========================================
* Configuration: {'height': 720, 'width': 1280, 'frame_rate': 29.97002997002997, 'format': 'yuv444p', 'encoder': 'h264_nvenc', 'encoder_format': 'yuv444p'}
* Destination: 720p_cuda.mp4
========================================
- Processed 450 frames.
- Elapsed: 0.8508033889999069 seconds.
CUDA (from CUDA Tensor)¶
[50]:
device = "cuda:0"
encode_config = {
"encoder": "h264_nvenc",
"encoder_format": "yuv444p",
"encoder_option": {"gpu": "0"},
"hw_accel": device,
}
result[2, 1] = test_encode(video.to(torch.device(device)), "720p_cuda_hw.mp4", **pict_config, **encode_config)
========================================
* Configuration: {'height': 720, 'width': 1280, 'frame_rate': 29.97002997002997, 'format': 'yuv444p', 'encoder': 'h264_nvenc', 'encoder_format': 'yuv444p', 'encoder_option': {'gpu': '0'}, 'hw_accel': 'cuda:0'}
* Destination: 720p_cuda_hw.mp4
========================================
- Processed 450 frames.
- Elapsed: 0.6384492569999338 seconds.
Encode MP4 - 1080P¶
We make the video with even larger.
[51]:
pict_config = {
"height": 1080,
"width": 1920,
"frame_rate": 30000/1001,
"format": "yuv444p",
}
video = get_data(**pict_config)
CPU¶
[52]:
encode_config = {
"encoder": "libx264",
"encoder_format": "yuv444p",
}
result[0, 2] = test_encode(video, "1080p_cpu.mp4", **pict_config, **encode_config)
========================================
* Configuration: {'height': 1080, 'width': 1920, 'frame_rate': 29.97002997002997, 'format': 'yuv444p', 'encoder': 'libx264', 'encoder_format': 'yuv444p'}
* Destination: 1080p_cpu.mp4
========================================
- Processed 450 frames.
- Elapsed: 27.020421489 seconds.
CUDA (from CPU Tensor)¶
[53]:
encode_config = {
"encoder": "h264_nvenc",
"encoder_format": "yuv444p",
}
result[1, 2] = test_encode(video, "1080p_cuda.mp4", **pict_config, **encode_config)
========================================
* Configuration: {'height': 1080, 'width': 1920, 'frame_rate': 29.97002997002997, 'format': 'yuv444p', 'encoder': 'h264_nvenc', 'encoder_format': 'yuv444p'}
* Destination: 1080p_cuda.mp4
========================================
- Processed 450 frames.
- Elapsed: 1.60377999800005 seconds.
CUDA (from CUDA Tensor)¶
[54]:
device = "cuda:0"
encode_config = {
"encoder": "h264_nvenc",
"encoder_format": "yuv444p",
"encoder_option": {"gpu": "0"},
"hw_accel": device,
}
result[2, 2] = test_encode(video.to(torch.device(device)), "1080p_cuda_hw.mp4", **pict_config, **encode_config)
========================================
* Configuration: {'height': 1080, 'width': 1920, 'frame_rate': 29.97002997002997, 'format': 'yuv444p', 'encoder': 'h264_nvenc', 'encoder_format': 'yuv444p', 'encoder_option': {'gpu': '0'}, 'hw_accel': 'cuda:0'}
* Destination: 1080p_cuda_hw.mp4
========================================
- Processed 450 frames.
- Elapsed: 1.4101193979998925 seconds.
Result¶
Here is the result.
[55]:
labels = ["CPU", "CUDA (from CPU Tensor)", "CUDA (from CUDA Tensor)"]
columns = ["360P", "720P", "1080P"]
res = pd.DataFrame(
result.numpy(),
index=labels,
columns=columns,
)
print(res)
360P 720P 1080P
CPU 3.280830 11.638768 27.020422
CUDA (from CPU Tensor) 0.342944 0.850803 1.603780
CUDA (from CUDA Tensor) 0.242452 0.638449 1.410119
[56]:
plt.plot(result.T)
plt.legend(labels)
plt.xticks([i for i in range(3)], columns)
plt.grid(visible=True, axis='y')
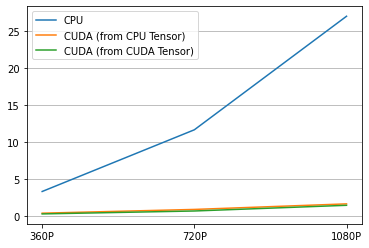
The resulting videos look like the following.
[61]:
from IPython.display import HTML
HTML('''
<div>
<video width=360 controls autoplay>
<source src="https://download.pytorch.org/torchaudio/tutorial-assets/streamwriter_360p_cpu.mp4" type="video/mp4">
</video>
<video width=360 controls autoplay>
<source src="https://download.pytorch.org/torchaudio/tutorial-assets/streamwriter_360p_cuda.mp4" type="video/mp4">
</video>
<video width=360 controls autoplay>
<source src="https://download.pytorch.org/torchaudio/tutorial-assets/streamwriter_360p_cuda_hw.mp4" type="video/mp4">
</video>
</div>
''')
[61]:
Conclusion¶
We looked at how to build FFmpeg libraries with NVDEC/NVENC support and use them from TorchAudio. NVDEC/NVENC provide significant speed up when saving/loading a video.